2018 Western New York Image and Signal Processing
Workshop
The Western New York Image and
Signal Processing Workshop (WNYISPW) is a venue for
promoting image and signal processing research in our area
and for facilitating interaction between academic
researchers, industry researchers, and students. The
workshop comprises both oral and poster presentations.
The workshop, building off of 20 successful years of the Western New York Image Processing Workshop (WNYIPW), is sponsored by the Rochester chapter of the IEEE Signal Processing Society with technical cooperation from the Rochester chapter of the Society for Imaging Science and Technology.
The workshop will be held on Friday, October 05, 2018, in Louise Slaughter Hall (Building SLA/078) at Rochester Institute of Technology in Rochester, NY.
Topics
Topics include, but are not limited to:
Important Dates
Dr. Simon Lucey, Associate Research Professor, Carnegie Mellon University
"How Do You Know What a Deep Network is Learning for a Vision Task?"
Abstract:
Modern deep learning algorithms are able to learn on training sets such that they achieve almost zero train error. What is all the more amazing, is that this performance tends to generalize well to unseen data - especially for visual detection and classification tasks. Increasingly, deep methods are being utilized in vision tasks such as object tracking and visual SLAM. These tasks differ fundamentally to traditional vision tasks where deep learning has been effective (e.g. object detection and classification) tasks as they are attempting to model the relative relationship between image frames. Although receiving state of the art performance on many benchmarks, it is easy to demonstrate empirically that deep methods are not always learning what we want them to learn for a given visual task - limiting their practical usage in real-world applications. In this talk we shall discuss recent advances my group has made towards making better guarantees over the generalization of deep learning methods for visual tasks where the relative relationship between images is important - most notably object tracking and VSLAM. In particular we shall discuss a new paradigm for efficient and generalizable object tracking which we refer to as Deep-LK. We shall also, discuss how these insights can be utilized in recent applications of deep learning to VSLAM. Finally, we will show some initial results on how geometric constraints can be elegantly combined with deep learning to further improve generalization performance.
Bio:
Simon Lucey (Ph.D.) is an associate research professor within the Robotics Institute at Carnegie Mellon University, where he is part of the Computer Vision Group, and leader of the CI2CV Laboratory. He is also a Senior Research Scientist at Argo AI (an autonomous vehicle startup based in Pittsburgh). Before returning to CMU he was a Principle Research Scientist at the CSIRO (Australia's premiere government science organization) for 5 years. A central goal of his research approach is to stay true, in terms of research and industry engagement, to the grand goals of computer vision as a scientific discipline. Specifically, he wants to draw inspiration from vision researchers of the past to attempt to unlock computational and mathematic models that underly the processes of visual perception.
Invited Speakers
Brad Palmer, Solutions Architect at NVIDIA Corporation
"End-to-end accelerated AI development"
Abstract:
Deep learning algorithms, accelerated with GPUs, are used extensively in many applications including image and signal processing. This talk will present the latest technology developments from NVIDIA and how they are being utilized, along with AI, to tackle challenges in image and signal processing. These technology developments include the use of GPUs, integrated hardware platforms, software libraries, SDKs, and optimizing compilers to accelerate AI from data ingest through training and inference.
Bio:
Bradley Palmer is a Solutions Architect at NVIDIA Corporation working with universities and research institutes to help them leverage NVIDIA’s technologies to accelerate their research. Bradley received an MSc degree in Electrical Engineering from Worcester Polytechnic Institute.
Dr. Emily Prud'hommeaux , Assistant Professor, Boston College
"Strengthening and Connecting Communities with Automatic Speech Recognition"
Abstract:
Recent increases in the accuracy of automatic speech recognition (ASR) systems have encouraged the widespread adoption of voice-based digital assistants and smart speakers. Although most of us use ASR to dictate text messages or issue commands to our devices, the technology offers utility beyond simply providing an alternative means of text entry. In this talk, I will describe how ASR can be deployed to support the transmission and preservation of information in underserved communities, including speakers of endangered languages and individuals facing communication challenges. After providing a brief overview of the ASR frameworks typically used for a language with substantial audio and text resources, such as standard American English, I will discuss methods for adapting these frameworks for use in low-resource scenarios. Finally, I will present the results of recent work using ASR to document Seneca, an indigenous language of Western New York.
Bio:
Emily Prud’hommeaux is an Assistant Professor in the Department of Computer Science at Boston College. Before joining BC, she was an Assistant Professor in the College of Liberal Arts at RIT, where she maintains a research appointment. Her research, currently supported by NSF, CRA, and NIH, focuses on natural language processing and speech signal processing for health and accessibility. She is co-founder of RIT’s Computational Linguistics and Speech Processing (CLaSP) lab, and she currently serves as Secretary-Treasurer of the ACL/ISCA Special Interest Group on Speech and Language Processing for Assistive Technologies.
Dr. Linwei Wang , Assistant Professor, Rochester Institute of Technology
"High-dimensional Bayesian Active Learning for Personalized Modeling"
Abstract:
Computer models of virtual organs, customized to an individual’s anatomy and physiology, are demonstrating increasing potential in improving personalized medicine. The estimation of patient-specific tissue properties in the form of model parameters, however, remains a significant challenge because of the complexity of the computer model and the high dimensionality of these model parameters. In this work, we present a novel approach that casts this estimation problem in the setting of active Bayesian learning, in which a Gaussian process surrogate of the objective function will be learned by actively selecting training points towards the optimum of the function. To address the challenge of active Bayesian learning over a high-dimensional (HD) parameter space, we present two alternative approaches: anatomy-based multi-scale approach in which the GP construction and optimization is done in a coarse-to-fine manner over the parameter space, and an alternative approach that embeds a generative model of the HD parameter into the active Bayesian learning process. These approaches are applied to the personalization of cardiac electrophysiological models in both synthetic and real-data experiments.
Bio:
Dr. Linwei Wang is an Associate Professor in the PhD Program of Computing and Information Sciences at the Rochester Institute of Technology in Rochester, NY. Her research interests center around large-scae statistical inference and its application to biomedical signal and image analysis, with a focus on improving patient care in cardiac arrhythmia and other heart diseases She currently directs the Computational Biomedical Lab at RIT. Her lab’s research is funded by the National Science Foundation and the National Institutes of Health. She is a recipient of the NSF CAREER Award in 2014. Dr. Wang obtained her bachelor degree in Optic-Electrical Engineering from Zhejiang University (China) in 2005, her master degree in Electronic and Computer Engineering from Hong Kong University of Science and Technology in 2007, and her PhD in Computing and Information Sciences from RIT prior to joining the faculty of RIT in 2009.
Dr. Chenliang Xu, Assistant Professor, University of Rochester
"Audio-Visual Scene Understanding"
Abstract:
Understanding scenes around us is a fundamental capability in human intelligence. Similarly, designing computer algorithms that can understand scenes is a fundamental problem in artificial intelligence. Humans consciously or unconsciously use all five senses (vision, audition, taste, smell, and touch) to understand a scene, as different senses provide complimentary information. Existing machine scene understanding algorithms, however, are designed to rely on just a single modality. Take the two most commonly used senses, vision and audition, as an example, there are scene understanding algorithms designed to deal with each single modality. However, no systematic investigations have been conducted to integrate these two modalities towards more comprehensive audio-visual scene understanding. Here, I will talk about two recent works from my group. The first work addresses the cross-modal audio-visual generation problem leveraging the power of deep generative adversarial training. We show state-of-the-art performance in constrained domains such as music performance and lip reading. The second work addresses the general scene audio-visual event localization. We show using both modalities cohesively in a deep time series model outperform using visual-alone or audio-alone.
Bio:
Chenliang Xu is an Assistant Professor in the Department of Computer Science at the University of Rochester. He received his Ph.D. degree from the University of Michigan in 2016, and MS degree from SUNY Buffalo in 2012, both in Computer Science. He received his BS degree in Information and Computing Science from Nanjing University of Aeronautics and Astronautics in 2010. His research interests include computer vision and its relations to natural language, robotics, and data science. His work primarily focuses on problems in video understanding such as video segmentation, activity recognition, and multimodal vision-and-x modeling. He is a recipient of NSF BIGDATA award 2017, University of Rochester AR/VR Pilot Award, and the best paper award at SMC 2017.
Dr. Richard Zanibbi, Professor, Rochester Institute of Technology
"Visual Search Engine for Math in Lecture Videos and LaTeX Notes"
Abstract:
To fill a gap in online educational tools, we are working to support search in lecture videos using formulas from lecture notes and vice versa. We convert a single-shot lecture video to a sequence of keyframes capturing whiteboard contents along with when they were written in the video. We then train classifiers for handwritten symbols using the CROHME dataset, and for typeset symbols in LaTeX using generated images. Symbols detected in video keyframes and LaTeX formula images are indexed using Line-of-Sight graphs. For search, we lookup pairs of symbols that can ‘see’ each other, and connected pairs are merged to identify the largest match within each indexed image. Matches are ranked using symbol probabilities and angles between symbol pairs. By combining our search engine (Tangent-V) with temporal keyframe metadata, we can navigate to where a query formula in LaTeX is first drawn in a lecture video. To use our technique in other domains, only the OCR modules require updating. Source code for our system is available (https://www.cs.rit.edu/~dprl/Software.html). [ Note: joint work with Dr. Kenny Davila (CUBS, University at Buffalo) ].
Bio:
Dr. Richard Zanibbi is a Professor of Computer Science at RIT, where he directs the Document and Pattern Recognition Lab (dprl). His research interests include document recognition, information retrieval, and human-computer interaction. Dr. Zanibbi received his PhD from Queen's University (Canada), and held an NSERC Postdoctoral Fellowship at the Centre for Pattern Recognition and Machine Learning (CENPARMI) in Montréal before coming to RIT. He was General Chair for the International Conference on Frontiers in Handwriting Recognition (ICFHR 2018) and SPIE Document Recognition and Retrieval (DRR 2012 and 2013). His research has been supported by the NSF, Xerox, Google, the French Government, and the Alfred P. Sloan Foundation.
Dr. Zhengwu Zhang, Assistant Professor, University of Rochester
"Geometric modeling of Brain Structural Connectome"
Abstract:
Advanced brain imaging techniques make it possible to measure individuals' structural connectomes in large cohort studies non-invasively. The structural connectome is initially shaped by genetics and subsequently refined by the environment. It is extremely interesting to study structural connectomes and their relationships to environmental factors or human traits, which motivates the routine collection of high-resolution connectomes in large human studies (e.g., the Human Connectome Project and UK Biobank). However, there is a fundamental gap between state-of-the-art in image acquisition and the tools available to reconstruct and analyze the connectome data, due to the complexity of data. In this talk, I will introduce a population-based structural connectome (PSC) mapping framework to reproducibly extract binary networks, weighted networks, and streamline-based brain connectomes. In addition, some novel statistical methods for analyzing the outputs of PSC and a user-friendly software package that implements the PSC will be introduced.
Bio:
My main interests lie in developing statistical methods for high-dimensional “objects” with low-dimensional underlying structures, e.g. images, contours, surfaces, networks and time-indexed paths on non-linear manifolds. These datatypes are abundant in various fields: neuroscience, epidemiology, genomics, meteorology and computer vision. Interdisciplinary thinking across different fields, e.g. Statistics, Computer Science, Mathematics, is the key to solve challenges raised from efficiently analyzing such "objects".
Dr. Christopher J. James, Professor, University of Warwick
"Independent Component Analysis in Brain Signals"
Abstract:
Independent Component Analysis (ICA) is a biomedical signal processing technique that has gained in popularity over the years used as a means of extracting meaningful information from a number of measurements made across the body. In Neural Engineering in particular, ICA has provided a very useful means of extracting information about neural sources, or otherwise, from recordings of electromagnetic brain signals (i.e. the electroencephalogram and magnetoencephalogram – EEG and MEG respectively). Inherently ICA is about the separation of statistically independent sources from a set of mixed measurements or recordings – for example in extracting eye-blinks from EEG or identifying ictal activity mixed in with ongoing EEG, etc. The strong assumption of statistical independence of the underlying sources is usually well met in neural engineering cases. The “default” methods of ICA usually work with a number of simultaneously measured channels and these report on a similar number of independent sources. In previous work we have shown, however, that ‘single-channel’ ICA is possible and is in itself a very powerful technique for the extraction of multiple (independent) sources underlying a single channel measurement – a very useful technique in applications that require ambulatory brain signal recordings. Whereas ‘standard’ ICA can be termed as ensemble or ‘spatial’ ICA, single channel ICA can be termed as ‘temporal’ ICA – as there is no spatial information informing the ICA process due to the single channel arrangement. The logical progression for ICA is to perform space-time (or spatio-temporal) ICA, whereby the ICA process is informed by means of both spatial and temporal/spectral information derived from a set of neural signal recordings. We can show that this, so called, space-time ICA results in a powerful algorithm that can extract meaningful information in brain signal recordings across a number of conditions. The technique is not without its issues, and suffers from the same problems standard ICA suffers from; these include issues around the assumptions of linear, noiseless, statistically independent mixing of sources as well as the dilemma of choosing sources of relevance once the ICA process is complete. With space-time ICA the problem is compounded somewhat due to the curse of dimensionality.
Bio:
Prof. Christopher J. James was appointed Professor of Healthcare Technology in 2010 and was founding Director of the Institute of Digital Healthcare at UoW. He now has a chair in Biomedical Engineering and is Director of Warwick Engineering in Biomedicine at the School of Engineering, UoW. Prof James’ research activity centres on the development of processing techniques applied to the analysis of the electromagnetic (EM) activity of the human brain, particularly in a functional neuroimaging context. Prof James has over 160 publications in varied biomedical engineering journals and refereed conferences. He is past Chairman of the Executive Committee of the Institution of Engineering and Technology (IET) Healthcare Technology Network and is on the Royal Academy of Engineering’s Panel for Biomedical Engineering. In 2013 he was awarded the IET Sir Monty Finniston Achievement Award for his work in the Biomedical Engineering field. He is founding Editor-in-Chief of the IET Healthcare Technology Letters Journal. Recent Research has been devoted to creating automated analysis techniques for the analysis of EEG data in epilepsy – these include automated spike and seizure detection algorithms, EEG (and MEG) de-noising algorithms and seizure onset prediction algorithms. Prof James is particularly interested in the development of techniques of Blind Source Separation (BSS) and Independent Component Analysis (ICA) for EM brain signal analysis such as denoising, source identification and extraction, and for the automation of such algorithms for clinical use. His work is applied to the biomedical fields of epilepsy research; Brain-Computer Interfacing; EEG denoising for evoked potentials analysis (BCI and use with Cochlear Implants); EEG/MEG slow-wave analysis for ADHD diagnosis and understanding; heart and lung sound detection and identification; pattern processing of electrophysiological signals from C. elegans as well as their behavioural monitoring through image processing. He has designed and distributes the TrakBox worm tracking platform used by a number of labs world-wide for worm behaviour monitoring.
Tutorials
Shounak Mitra, Technical Product Marketing Manager
Balaji Sharma, Customer Success Engineer
Tim Mathieu, Field Engineer and Account Manager
"Demystifying deep learning: A practical approach in MATLAB"
Abstract:
Are you new to deep learning and want to learn how to use it in your work? Deep learning can achieve state-of-the-art accuracy in many humanlike tasks such as naming objects in a scene or recognizing optimal paths in an environment.
The main tasks are to assemble large data sets, create a neural network, to train, visualize, and evaluate different models, using specialized hardware - often requiring unique programming knowledge. These tasks are frequently even more challenging because of the complex theory behind them.
In this session, we’ll demonstrate new MATLAB features that simplify these tasks and eliminate the low-level programming. In doing so, we’ll decipher practical knowledge of the domain of deep learning. We’ll build and train neural networks that recognize handwriting and classify food in a scene.
Along the way, you’ll see MATLAB features that make it easy to:
Notes: This is a seminar style presentation and there is no prior preparation from the attendees.
Paper Submission
The Call for Papers can be found here.
Paper submission is now closed! Paper submission deadline 11:59pm EDT on September 14, 2018.
--> Prospective authors are invited to submit a 4-page paper + 5th page of references here: https://cmt3.research.microsoft.com/WNYISPW2018/
Authors should use the same formatting/templates described in the ICIP 2015 Paper Kit.
All accepted papers will be submitted to IEEE Xplore and EI. Past WNYIPW and WNYISPW proceedings can be found here:
Prospective authors are invited to submit an abstract here: https://cmt3.research.microsoft.com/WNYISPW2018/
Awards
To encourage student participation, a best student paper and best student poster
award will be given.
If your department does not have poster printing capabilities, you can get posters printed at "the Hub Express" in the RIT Student Union, hubxpr@rit.edu, 585-475-3471. Color wide format inkjet is $7/sq.ft. Mounting (up to 30x40) is $4/sq.ft. (Contact the Hub Express if you have larger than 30x40)
Parking Instructions
Any non-RIT attendees are allowed to park in either Lot T or
the Global Village Lot and then walk to Louise Slaughter
Hall (SLA Building). See the
campus map with parking information (you need to print out a parking pass and
place on your windshield). If you forget to print out a permit,
Non-RIT visitors can stop by the RIT Welcome Center (flagpole entrance) on the
day of the Workshop to get a parking pass.
The 2018 WNYISPW was held on Oct 5, 2018. Thanks to David Odgers, pictures can be found here.
Detailed Schedule follows
The workshop, building off of 20 successful years of the Western New York Image Processing Workshop (WNYIPW), is sponsored by the Rochester chapter of the IEEE Signal Processing Society with technical cooperation from the Rochester chapter of the Society for Imaging Science and Technology.
The workshop will be held on Friday, October 05, 2018, in Louise Slaughter Hall (Building SLA/078) at Rochester Institute of Technology in Rochester, NY.
Topics
Topics include, but are not limited to:- Formation, Processing, and/or Analysis of Signals, Images, or Video
- Computer Vision
- Information Retrieval
- Image and Color Science
- Applications of Image and Signal Processing, including:
- Medical Image and Signal Analysis
- Audio Processing and Analysis
- Remote Sensing
- Archival Imaging
- Printing
- Consumer Devices
- Security
- Surveillance
- Document Imaging
- Art Restoration and Analysis
- Astronomy
Important Dates
Paper submission opens: | August 13, 2018 |
Paper submission closes: | September 14, 2018 |
Notification of Acceptance: | September 24, 2018 |
Early (online) registration deadline: | September 21, 2018 |
Submission of camera-ready paper: | October 8, 2018 |
Workshop: | October 05, 2018 |
Keynote Speakers
We are happy to announce our keynote speaker:Dr. Simon Lucey, Associate Research Professor, Carnegie Mellon University
"How Do You Know What a Deep Network is Learning for a Vision Task?"
Abstract:
Modern deep learning algorithms are able to learn on training sets such that they achieve almost zero train error. What is all the more amazing, is that this performance tends to generalize well to unseen data - especially for visual detection and classification tasks. Increasingly, deep methods are being utilized in vision tasks such as object tracking and visual SLAM. These tasks differ fundamentally to traditional vision tasks where deep learning has been effective (e.g. object detection and classification) tasks as they are attempting to model the relative relationship between image frames. Although receiving state of the art performance on many benchmarks, it is easy to demonstrate empirically that deep methods are not always learning what we want them to learn for a given visual task - limiting their practical usage in real-world applications. In this talk we shall discuss recent advances my group has made towards making better guarantees over the generalization of deep learning methods for visual tasks where the relative relationship between images is important - most notably object tracking and VSLAM. In particular we shall discuss a new paradigm for efficient and generalizable object tracking which we refer to as Deep-LK. We shall also, discuss how these insights can be utilized in recent applications of deep learning to VSLAM. Finally, we will show some initial results on how geometric constraints can be elegantly combined with deep learning to further improve generalization performance.
Bio:
Simon Lucey (Ph.D.) is an associate research professor within the Robotics Institute at Carnegie Mellon University, where he is part of the Computer Vision Group, and leader of the CI2CV Laboratory. He is also a Senior Research Scientist at Argo AI (an autonomous vehicle startup based in Pittsburgh). Before returning to CMU he was a Principle Research Scientist at the CSIRO (Australia's premiere government science organization) for 5 years. A central goal of his research approach is to stay true, in terms of research and industry engagement, to the grand goals of computer vision as a scientific discipline. Specifically, he wants to draw inspiration from vision researchers of the past to attempt to unlock computational and mathematic models that underly the processes of visual perception.
Invited Speakers
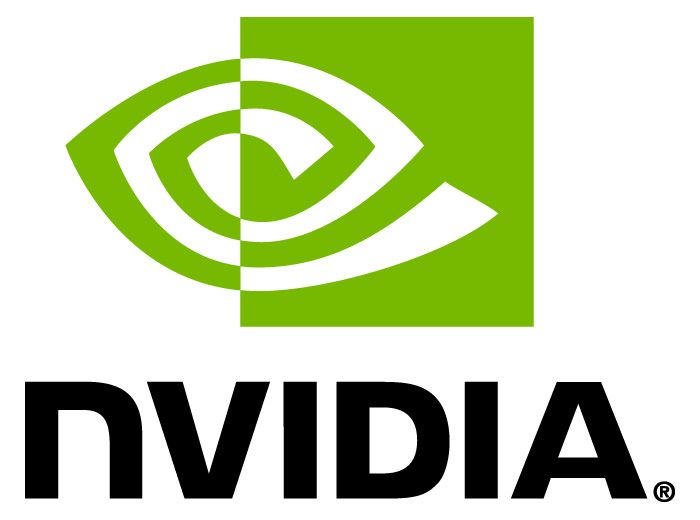
"End-to-end accelerated AI development"
Abstract:
Deep learning algorithms, accelerated with GPUs, are used extensively in many applications including image and signal processing. This talk will present the latest technology developments from NVIDIA and how they are being utilized, along with AI, to tackle challenges in image and signal processing. These technology developments include the use of GPUs, integrated hardware platforms, software libraries, SDKs, and optimizing compilers to accelerate AI from data ingest through training and inference.
Bio:
Bradley Palmer is a Solutions Architect at NVIDIA Corporation working with universities and research institutes to help them leverage NVIDIA’s technologies to accelerate their research. Bradley received an MSc degree in Electrical Engineering from Worcester Polytechnic Institute.
Dr. Emily Prud'hommeaux , Assistant Professor, Boston College
"Strengthening and Connecting Communities with Automatic Speech Recognition"
Abstract:
Recent increases in the accuracy of automatic speech recognition (ASR) systems have encouraged the widespread adoption of voice-based digital assistants and smart speakers. Although most of us use ASR to dictate text messages or issue commands to our devices, the technology offers utility beyond simply providing an alternative means of text entry. In this talk, I will describe how ASR can be deployed to support the transmission and preservation of information in underserved communities, including speakers of endangered languages and individuals facing communication challenges. After providing a brief overview of the ASR frameworks typically used for a language with substantial audio and text resources, such as standard American English, I will discuss methods for adapting these frameworks for use in low-resource scenarios. Finally, I will present the results of recent work using ASR to document Seneca, an indigenous language of Western New York.
Bio:
Emily Prud’hommeaux is an Assistant Professor in the Department of Computer Science at Boston College. Before joining BC, she was an Assistant Professor in the College of Liberal Arts at RIT, where she maintains a research appointment. Her research, currently supported by NSF, CRA, and NIH, focuses on natural language processing and speech signal processing for health and accessibility. She is co-founder of RIT’s Computational Linguistics and Speech Processing (CLaSP) lab, and she currently serves as Secretary-Treasurer of the ACL/ISCA Special Interest Group on Speech and Language Processing for Assistive Technologies.
Dr. Linwei Wang , Assistant Professor, Rochester Institute of Technology
"High-dimensional Bayesian Active Learning for Personalized Modeling"
Abstract:
Computer models of virtual organs, customized to an individual’s anatomy and physiology, are demonstrating increasing potential in improving personalized medicine. The estimation of patient-specific tissue properties in the form of model parameters, however, remains a significant challenge because of the complexity of the computer model and the high dimensionality of these model parameters. In this work, we present a novel approach that casts this estimation problem in the setting of active Bayesian learning, in which a Gaussian process surrogate of the objective function will be learned by actively selecting training points towards the optimum of the function. To address the challenge of active Bayesian learning over a high-dimensional (HD) parameter space, we present two alternative approaches: anatomy-based multi-scale approach in which the GP construction and optimization is done in a coarse-to-fine manner over the parameter space, and an alternative approach that embeds a generative model of the HD parameter into the active Bayesian learning process. These approaches are applied to the personalization of cardiac electrophysiological models in both synthetic and real-data experiments.
Bio:
Dr. Linwei Wang is an Associate Professor in the PhD Program of Computing and Information Sciences at the Rochester Institute of Technology in Rochester, NY. Her research interests center around large-scae statistical inference and its application to biomedical signal and image analysis, with a focus on improving patient care in cardiac arrhythmia and other heart diseases She currently directs the Computational Biomedical Lab at RIT. Her lab’s research is funded by the National Science Foundation and the National Institutes of Health. She is a recipient of the NSF CAREER Award in 2014. Dr. Wang obtained her bachelor degree in Optic-Electrical Engineering from Zhejiang University (China) in 2005, her master degree in Electronic and Computer Engineering from Hong Kong University of Science and Technology in 2007, and her PhD in Computing and Information Sciences from RIT prior to joining the faculty of RIT in 2009.
Dr. Chenliang Xu, Assistant Professor, University of Rochester
"Audio-Visual Scene Understanding"
Abstract:
Understanding scenes around us is a fundamental capability in human intelligence. Similarly, designing computer algorithms that can understand scenes is a fundamental problem in artificial intelligence. Humans consciously or unconsciously use all five senses (vision, audition, taste, smell, and touch) to understand a scene, as different senses provide complimentary information. Existing machine scene understanding algorithms, however, are designed to rely on just a single modality. Take the two most commonly used senses, vision and audition, as an example, there are scene understanding algorithms designed to deal with each single modality. However, no systematic investigations have been conducted to integrate these two modalities towards more comprehensive audio-visual scene understanding. Here, I will talk about two recent works from my group. The first work addresses the cross-modal audio-visual generation problem leveraging the power of deep generative adversarial training. We show state-of-the-art performance in constrained domains such as music performance and lip reading. The second work addresses the general scene audio-visual event localization. We show using both modalities cohesively in a deep time series model outperform using visual-alone or audio-alone.
Bio:
Chenliang Xu is an Assistant Professor in the Department of Computer Science at the University of Rochester. He received his Ph.D. degree from the University of Michigan in 2016, and MS degree from SUNY Buffalo in 2012, both in Computer Science. He received his BS degree in Information and Computing Science from Nanjing University of Aeronautics and Astronautics in 2010. His research interests include computer vision and its relations to natural language, robotics, and data science. His work primarily focuses on problems in video understanding such as video segmentation, activity recognition, and multimodal vision-and-x modeling. He is a recipient of NSF BIGDATA award 2017, University of Rochester AR/VR Pilot Award, and the best paper award at SMC 2017.
Dr. Richard Zanibbi, Professor, Rochester Institute of Technology
"Visual Search Engine for Math in Lecture Videos and LaTeX Notes"
Abstract:
To fill a gap in online educational tools, we are working to support search in lecture videos using formulas from lecture notes and vice versa. We convert a single-shot lecture video to a sequence of keyframes capturing whiteboard contents along with when they were written in the video. We then train classifiers for handwritten symbols using the CROHME dataset, and for typeset symbols in LaTeX using generated images. Symbols detected in video keyframes and LaTeX formula images are indexed using Line-of-Sight graphs. For search, we lookup pairs of symbols that can ‘see’ each other, and connected pairs are merged to identify the largest match within each indexed image. Matches are ranked using symbol probabilities and angles between symbol pairs. By combining our search engine (Tangent-V) with temporal keyframe metadata, we can navigate to where a query formula in LaTeX is first drawn in a lecture video. To use our technique in other domains, only the OCR modules require updating. Source code for our system is available (https://www.cs.rit.edu/~dprl/Software.html). [ Note: joint work with Dr. Kenny Davila (CUBS, University at Buffalo) ].
Bio:
Dr. Richard Zanibbi is a Professor of Computer Science at RIT, where he directs the Document and Pattern Recognition Lab (dprl). His research interests include document recognition, information retrieval, and human-computer interaction. Dr. Zanibbi received his PhD from Queen's University (Canada), and held an NSERC Postdoctoral Fellowship at the Centre for Pattern Recognition and Machine Learning (CENPARMI) in Montréal before coming to RIT. He was General Chair for the International Conference on Frontiers in Handwriting Recognition (ICFHR 2018) and SPIE Document Recognition and Retrieval (DRR 2012 and 2013). His research has been supported by the NSF, Xerox, Google, the French Government, and the Alfred P. Sloan Foundation.
Dr. Zhengwu Zhang, Assistant Professor, University of Rochester
"Geometric modeling of Brain Structural Connectome"
Abstract:
Advanced brain imaging techniques make it possible to measure individuals' structural connectomes in large cohort studies non-invasively. The structural connectome is initially shaped by genetics and subsequently refined by the environment. It is extremely interesting to study structural connectomes and their relationships to environmental factors or human traits, which motivates the routine collection of high-resolution connectomes in large human studies (e.g., the Human Connectome Project and UK Biobank). However, there is a fundamental gap between state-of-the-art in image acquisition and the tools available to reconstruct and analyze the connectome data, due to the complexity of data. In this talk, I will introduce a population-based structural connectome (PSC) mapping framework to reproducibly extract binary networks, weighted networks, and streamline-based brain connectomes. In addition, some novel statistical methods for analyzing the outputs of PSC and a user-friendly software package that implements the PSC will be introduced.
Bio:
My main interests lie in developing statistical methods for high-dimensional “objects” with low-dimensional underlying structures, e.g. images, contours, surfaces, networks and time-indexed paths on non-linear manifolds. These datatypes are abundant in various fields: neuroscience, epidemiology, genomics, meteorology and computer vision. Interdisciplinary thinking across different fields, e.g. Statistics, Computer Science, Mathematics, is the key to solve challenges raised from efficiently analyzing such "objects".
Dr. Christopher J. James, Professor, University of Warwick
"Independent Component Analysis in Brain Signals"
Abstract:
Independent Component Analysis (ICA) is a biomedical signal processing technique that has gained in popularity over the years used as a means of extracting meaningful information from a number of measurements made across the body. In Neural Engineering in particular, ICA has provided a very useful means of extracting information about neural sources, or otherwise, from recordings of electromagnetic brain signals (i.e. the electroencephalogram and magnetoencephalogram – EEG and MEG respectively). Inherently ICA is about the separation of statistically independent sources from a set of mixed measurements or recordings – for example in extracting eye-blinks from EEG or identifying ictal activity mixed in with ongoing EEG, etc. The strong assumption of statistical independence of the underlying sources is usually well met in neural engineering cases. The “default” methods of ICA usually work with a number of simultaneously measured channels and these report on a similar number of independent sources. In previous work we have shown, however, that ‘single-channel’ ICA is possible and is in itself a very powerful technique for the extraction of multiple (independent) sources underlying a single channel measurement – a very useful technique in applications that require ambulatory brain signal recordings. Whereas ‘standard’ ICA can be termed as ensemble or ‘spatial’ ICA, single channel ICA can be termed as ‘temporal’ ICA – as there is no spatial information informing the ICA process due to the single channel arrangement. The logical progression for ICA is to perform space-time (or spatio-temporal) ICA, whereby the ICA process is informed by means of both spatial and temporal/spectral information derived from a set of neural signal recordings. We can show that this, so called, space-time ICA results in a powerful algorithm that can extract meaningful information in brain signal recordings across a number of conditions. The technique is not without its issues, and suffers from the same problems standard ICA suffers from; these include issues around the assumptions of linear, noiseless, statistically independent mixing of sources as well as the dilemma of choosing sources of relevance once the ICA process is complete. With space-time ICA the problem is compounded somewhat due to the curse of dimensionality.
Bio:
Prof. Christopher J. James was appointed Professor of Healthcare Technology in 2010 and was founding Director of the Institute of Digital Healthcare at UoW. He now has a chair in Biomedical Engineering and is Director of Warwick Engineering in Biomedicine at the School of Engineering, UoW. Prof James’ research activity centres on the development of processing techniques applied to the analysis of the electromagnetic (EM) activity of the human brain, particularly in a functional neuroimaging context. Prof James has over 160 publications in varied biomedical engineering journals and refereed conferences. He is past Chairman of the Executive Committee of the Institution of Engineering and Technology (IET) Healthcare Technology Network and is on the Royal Academy of Engineering’s Panel for Biomedical Engineering. In 2013 he was awarded the IET Sir Monty Finniston Achievement Award for his work in the Biomedical Engineering field. He is founding Editor-in-Chief of the IET Healthcare Technology Letters Journal. Recent Research has been devoted to creating automated analysis techniques for the analysis of EEG data in epilepsy – these include automated spike and seizure detection algorithms, EEG (and MEG) de-noising algorithms and seizure onset prediction algorithms. Prof James is particularly interested in the development of techniques of Blind Source Separation (BSS) and Independent Component Analysis (ICA) for EM brain signal analysis such as denoising, source identification and extraction, and for the automation of such algorithms for clinical use. His work is applied to the biomedical fields of epilepsy research; Brain-Computer Interfacing; EEG denoising for evoked potentials analysis (BCI and use with Cochlear Implants); EEG/MEG slow-wave analysis for ADHD diagnosis and understanding; heart and lung sound detection and identification; pattern processing of electrophysiological signals from C. elegans as well as their behavioural monitoring through image processing. He has designed and distributes the TrakBox worm tracking platform used by a number of labs world-wide for worm behaviour monitoring.
Tutorials
Shounak Mitra, Technical Product Marketing ManagerBalaji Sharma, Customer Success Engineer
Tim Mathieu, Field Engineer and Account Manager
"Demystifying deep learning: A practical approach in MATLAB"
Abstract:
Are you new to deep learning and want to learn how to use it in your work? Deep learning can achieve state-of-the-art accuracy in many humanlike tasks such as naming objects in a scene or recognizing optimal paths in an environment.
The main tasks are to assemble large data sets, create a neural network, to train, visualize, and evaluate different models, using specialized hardware - often requiring unique programming knowledge. These tasks are frequently even more challenging because of the complex theory behind them.
In this session, we’ll demonstrate new MATLAB features that simplify these tasks and eliminate the low-level programming. In doing so, we’ll decipher practical knowledge of the domain of deep learning. We’ll build and train neural networks that recognize handwriting and classify food in a scene.
Along the way, you’ll see MATLAB features that make it easy to:
- Manage extremely large sets of images
- Perform classification and pixel-level semantic segmentation on images
- Import training data sets from networks such as GoogLeNet
- Automatically convert a model to CUDA to run on GPUs
Notes: This is a seminar style presentation and there is no prior preparation from the attendees.
Paper Submission
The Call for Papers can be found here.
Paper submission is now closed! Paper submission deadline 11:59pm EDT on September 14, 2018.
--> Prospective authors are invited to submit a 4-page paper + 5th page of references here: https://cmt3.research.microsoft.com/WNYISPW2018/
Authors should use the same formatting/templates described in the ICIP 2015 Paper Kit.
All accepted papers will be submitted to IEEE Xplore and EI. Past WNYIPW and WNYISPW proceedings can be found here:
Poster Submission
Authors who only want to be considered for a poster presentaiton have the option to submit an abstract in place of a full paper. (Note: Abstract-only submissions will not be searchable on IEEE Xplore)Prospective authors are invited to submit an abstract here: https://cmt3.research.microsoft.com/WNYISPW2018/
Author Attendance
At least one author of each accepted paper or poster must register and attend the workshop to give an oral or poster presentation. Failure to present the paper will result in automatic withdrawal of the paper from being published in the proceedings.Distant Authors
We will do our best to give early notification to those authors who need to make travel plans. No invitations will be given for international visas. At least one author needs to be present to prevent paper withdrawal from proceedings.Awards
To encourage student participation, a best student paper and best student poster
award will be given. Registration
Registration is available online here. Onsite registration will be also available, with onsite registration fees payable by cash or check. Fees enable attendance to all sessions and include breakfast, lunch, and afternoon snack. Registration fees are:- General Registration: $60 (with online registration by
09/21), $70 (online after 11/9 or onsite)
- Student Registration: $40 (with online registration by 09/21), $50 (online after 11/9 or onsite)
- IEEE or IS&T Membership: $40 (with online registration by 09/21), $50 (online after 11/9 or onsite)
- IEEE or IS&T Student Membership: $30 (with online registration by 09/21), $40 (online after 11/9 or onsite)
Conference at a Glance (Detailed Schedule below)
- 8:30-8:55am, Registration, breakfast
- 9am-12:40pm, Oral presentations
- 10:40-12:40am, Mathworks Deep Learning Tutorial
- 12:40-2pm, Lunch and posters
- 2-3pm, Keynote
- 3:15-5:15pm, Oral presentations
- 5:15pm, Awards
Oral Presentation Instructions
All oral presentations will be 12 minutes long plus 2 minutes for questions. Presentors supply their own laptop with a VGA/HDMI connector. Morning/afternoon presentors should test their laptop on the display screen during the 8:15-8:45am or 12:45-1:45pm timeframes respectively. Papers whose first author is a student qualify for best paper award.Poster Presentation Instructions
All printed posters must be no larger than 40" wide x 48" tall. Poster stations will be available for both mounted and unmounted posters. (If you can bring a mounted poster, please do so.) Attachment materials will be provided. All posters must be displayed by 11am and removed by 5:30pm. There are no electrical outlets next to the poster displays. Posters whose first author is a student qualify for best poster award.If your department does not have poster printing capabilities, you can get posters printed at "the Hub Express" in the RIT Student Union, hubxpr@rit.edu, 585-475-3471. Color wide format inkjet is $7/sq.ft. Mounting (up to 30x40) is $4/sq.ft. (Contact the Hub Express if you have larger than 30x40)
Parking Instructions
Any non-RIT attendees are allowed to park in either Lot T or
the Global Village Lot and then walk to Louise Slaughter
Hall (SLA Building). See the
campus map with parking information (you need to print out a parking pass and
place on your windshield). If you forget to print out a permit,
Non-RIT visitors can stop by the RIT Welcome Center (flagpole entrance) on the
day of the Workshop to get a parking pass.The 2018 WNYISPW was held on Oct 5, 2018. Thanks to David Odgers, pictures can be found here.
Detailed Schedule follows
- 8:30-8:55am, Registration, breakfast (Rooms 2210-2240)
- 8:55-9am, Welcome by Conference Chair (Rooms 2210-2240)
- 9:00am: Invited talk: Zhengwu Zhang, Assistant Professor, University of Rochester -
- 9:30am: Invited talk: Linwei Wang, Assistant Professor, Rochester Institute of Technology
- 10:00am: Invited talk: Brad Palmer, Solutions Architect, NVIDIA Corporation
- 10:30am: AM break
- 10:40am: Invited talk: Emily Prud’hommeaux, Assistant Professor, Boston College
- 11:10am: Invited talk: Christopher James, Professor, University of Warwick
- 11:40am-12:40pm: Oral papers (Rooms 2210-2240)
- “(Peak) Wall Stress as an Indicator of Abdominal Aortic Aneurysm Severity”, Golnaz Jalalahmadi; Cristian Linte
- “A Modified U-Net Convolutional Network Featuring a Nearest-neighbor Re-sampling-based Elastic-Transformation for Brain Tissue Characterization and Segmentation”, S. M. Kamrul Hasan; Cristian Linte --Runner up best paper award!
- “Destination-Directed Trajectory Modeling and Prediction Using Conditionally Markov Sequences”, Reza Rezaie; X. Rong Li
- “Faster Art-CNN: An Extremely Fast Style Transfer Network”, Bryan M Blakeslee; Raymond Ptucha; Andreas Savakis --Best paper award!
- 10:40am-12:40pm, MathWorks Tutorial (Room 2120): “Deep Learning for Image Processing & Computer Vision”
- Shounak Mitra, Technical Product Marketing Manager
- Balaji Sharma, Customer Success Engineer
- Tim Mathieu, MathWorks Field Engineer
- 12:40-2pm, Lunch and poster displays (Rooms 2210-2240, overflow 2140)
- “An Incoherent Receiver for Logarithmic Warped Frequency Domain Ruler Waveforms”, Alex N Byrley; Adly Fam
- “Nonsingular Gaussian Conditionally Markov Sequences”, Reza Rezaie; X. Rong Li
- “TallyQA: Answering Complex Counting Questions”, Manoj Acharya
- “Contemporary Multimodal Data Collection Methodology for Reliable Inference of Authentic Surprise”, Jordan E Shea; Cecilia Alm; Reynold Bailey
- “A Comparative Analysis of VQA algorithms on Natural and Synthetic Datasets”, Robik S Shrestha
- “Evaluating Bounded Classification Methods for Deep Neural Networks”, Ryne P Roady; Ronald Kemker; Ayesha Gonzales; Christopher Kanan
- “Explicitly Sample-Equivalent Dynamic Models for Gaussian Markov, Reciprocal, and Conditionally Markov Sequences”, Reza Rezaie; X. Rong Li
- “Memory Efficient Experience Replay for Streaming Learning”, Tyler Hayes; Nathan Cahill; Christopher Kanan
- “Fast Convolutional Neural Network Architectures for Very High Resolution Imagery” , Frank C Cwitkowitz; Christopher Kanan --Best poster award!
- “Real-Time Scale-Invariant License Plate Detection Using Cascade Classifiers”, Elnaz Yousefi; Amir Hossein Nazem Deligani; Jafar Jafari Amirbandi
- “Applying Hierarchical Contextual Parsing to Recognize Isolated Typeset Math Formula”, Mahshad Mahdavi; Richard Zanibbi --Runner up best poster award!
- “Symbolic Understanding of Anime with Deep Learning”, Tushar Sharma
- “GUI based Control Scheme for Ball-on-Plate System using Computer Vision”, Akanksh Kasula; Palash Thakur; Mukund Kumar Menon
- “Blind Identification of Invertible Graph Filters with Multiple Sparse Inputs”, Chang Ye; Rasoul Shafipour; Gonzalo Mateos
- “A Digraph Fourier Transform”, Rasoul Shafipour; Ali Khodabakhsh; Gonzalo Mateos; Evdokia Nikolova
- “Event level cross-category metric for evaluating gaze event classification”, Zhizhuo Yang; Rakshit S Kothari
- “Gaze in wild: A dataset for studying Vestibular-Ocular coordination in naturalistic tasks”, Rakshit S Kothari; Zhizhuo Yang; Jeff Pelz; Reynold Bailey; Christopher Kanan; Gabe Diaz
- “Pre-trained Deep Learning Algorithms for Detection and Classification of Diabetic Retinopathy: Review and Side-by-Side Comparison”, Roshan Reddy Upendra; Cristian Linte
- “On the Effect of Face Detection on Heart Rate Estimation in Videoplethysmography”, Ruslan Dautov; Celal Savur; Gill Tsouri
- “Gait Recognition Based on Tensor Analysis of Acceleration Data from Wearable Sensors”, Kshitij V Bichave; Owen Brewer; Max Gusinov; Panagiotis Markopoulos; Ivan Puchades --Runner up best poster award!
- “DVQA: Understanding Data Visualizations via Question Answering”, Kushal Kafle; Christopher Kanan; Scott Cohen; Brian Price
- “Improving ASR output for endangered language documentation”, Robert Jimerson
- 2-3pm, Keynote: Simon Lucey, Associate Research Professor, Carnegie Mellon University
- 2:50pm: PM break
- 3:15pm: Invited talk: Richard Zanibbi, Professor, Rochester Institute of Technology
- 3:45pm: Invited talk: Chenliang Xu, Assistant Professor, University of Rochester
- 4:15pm-5:15pm: Oral papers (Rooms 2210-2240)
- “Left Ventricular Ejection Fraction: Comparison between True Volume-based Measurements and Area-based Estimates”, Dawei Liu; isabelle Peck; Shusil Dangi; Karl Schwarz; Cristian Linte
- “Incremental Complex L1-PCA for Direction-of-Arrival Estimation”, Mayur Dhanaraj; Dimitris Chachlakis; Panos Markopoulos
- “Understanding Feature Discovery in Website Fingerprinting Attacks”, Nate J Mathews; Payap Sirinam; Matthew Wright
- “A Deeper Understanding of Luminance and Chrominance for Computer Vision”, Thomas B Kinsman
- 5:15pm, Closing and Awards (Rooms 2210-2240)
- Ziya Arnavut, SUNY Fredonia
- Nathan Cahill, Rochester Institute of Technology
- Zhiyao Duan, University of Rochester
- Christopher Kanan, Rochester Institute of Technology
- Paul Lee, University of Rochester
- Cristian Linte, Rochester Institute of Technology
- David Odgers, Odgers Imaging
- Raymond Ptucha, Rochester Institute of Technology
- Richard Zanibbi, Rochester Institute of Technology