Gold Sponsors |
|
|
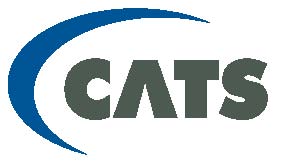  |
|
|
| Tutorial: Rapid Realization of Diagnostics and Prognostics
Date: Saturday, August 21, 2010
Time: 8:30 – 12:00 pm
Tutorial Speakers:
1. Canh Ly, Ph.D., Senior Researcher, U.S. Army Research Laboratory, MD, USA
2. Jay Lee, Professor, Ohio Eminent Scholar and Director of NSF I/UCRC on Intelligent
Maintenance Systems (IMS), University of Cincinnati, Ohio, USA
3. David Siegel, PhD Student, IMS Center, University of Cincinnati, Ohio, USA
Tutorial Description:
This tutorial is intended for researchers and practitioners who want to track new developments in Sensor Networks for Automation Applications but do not have the time or patience to read relevant papers or specifications. The tutorial will serve extremely useful for research students who are looking for open problems in this space and who are keen to build a deep understanding in the area. Managers wishing to obtain a better understanding of the application space will also benefit from this tutorial.
Tutorial Objectives:
The tutorial gives an introduction about the current state-of-the-art practices in machine prognostics and diagnostics design and implementation. A systematic methodology and tools for enabling rapid realization of prognostic and diagnostic solutions will be discussed with case studies.
Currently, diagnostics and prognostics development is primary based on a trial-and-error approach for specific applications. It lacks systematic methodology to enable the rapid development and validation of the tools. What is needed is a systematic approach and a developed toolbox of algorithms that are ready to be deployed, to allow for more expedite development of prognostic and health management solutions that can leverage the previous knowledge base and approach of successful case studies as well as a full set of tools that the user can select based on the application and particular problem.
The tutorial presents a systematic methodology for developing prognostic and health management solutions, as well as a set of algorithms for feature extraction, machine health assessment and health prediction. Further this overall approach and developed tools are demonstrated in selected case studies including military, manufacturing, and energy applications.
Topics covered in the tutorial:
- Review of current practices in research in this area
- Motivation and Need for Systematic Methodology for Prognostics and Health Management (PHM)
- Overview and goals of the tutorial
- Systematic Approach for PHM Design and Implementation
- Review Relevant algorithms and tools
- Demonstrate approach and tools with various successful case studies in military, manufacturing, and energy domains/industries
- Systematic Approach for PHM Design and Implementation
- IMS Approach
- Critical Asset / Critical Component Selection
- 5S Approach (Streamline, Smart Processing, Synchronize, Standardize, Sustain)
- Strategic Data Management
- Algorithm Selection Based on Application Domain
- Signal Processing & Feature Extraction
- Review of various signal processing techniques, data transforms, and generic and specific feature extraction and selection routines
- Heath Assessment
- Review of different health assessment algorithms including regression based, distance based, neural network pattern recognition methods
- Anomaly detection, weakness detection for fleet of units
- Performance Prediction
- Time Series Prediction Methods
- Statistical models for RUL estimation
- Similarity/instance based Remain Useful Life (RUL) estimation
- Case Studies
- Non-industrial applications: Aviation, ground and other systems
- Manufacturing applications
- Renewable energy: wind turbine
- Conclusions and Future Direction of PHM
Tutorial Agenda:
08:30-09:10 |
Introduction: The needs for Conditioned-base maintenance Plus (CBM+) and the thrust for Rapid Realization of Diagnostics and Prognostics for non-industrial perspectives – Canh Ly |
09:10-09:50 |
What is Rapid Realization of Diagnostics and Prognostics for industrial and manufacturing perspectives? Overview of Systematic Approach for Prognostic Health Management (PHM) Design and Implementations – Jay Lee |
09:50-10:00 |
Break |
10:00-11:30 |
Review relevant algorithms and tools. Demonstrate the systematic approach and tools with various successful case studies – David Siegel |
11:30-12:00 |
Overview/Discussion on Case studies / Discussion of Future Direction of PHM – All |
Speakers’ Biographies:
Dr. Canh Ly
Senior Researcher, U.S. Army Research Laboratory, Maryland, USA)
(canh.ly@us.army.mil, 301-394-0868)
Dr. Canh Ly received the Bachelor of Science degree in Electrical Engineering from the University of Maryland, College Park, MD, the Master of Science degree in Electrical Engineering from the Johns Hopkins University, and the Ph.D. degree in Information Technology from the George Mason University. Dr. Ly has been a Senior Member of Technical Staff at U.S. Army Research Laboratory (ARL). He has submitted three patents related to frequency estimation technique, patch array antennas, and published papers in IEEE Radar Conference Proceedings, Asilomar Conference, and SPIE Conference. His research interests include developing algorithms for fault diagnosis and failure prognosis, antenna design, superresolution algorithms for millimeter wave (MMW) radars, statistical signal processing, advanced HEMP techniques, and radar array processing.
Professor Jay Lee
Ohio Eminent Scholar and Director of NSF I/UCRC on Intelligent Maintenance Systems (IMS), University of Cincinnati, Ohio, USA)
(jay.lee@uc.edu)
Dr. Jay Lee is Ohio Eminent Scholar and L.W. Scott Alter Chair Professor at the Univ. of Cincinnati and is founding director of National Science Foundation (NSF) Industry/University Cooperative Research Center (I/UCRC) on Intelligent Maintenance Systems (IMS www.imscenter.net ) which is a multi-campus NSF Center of Excellence between the Univ. of Cincinnati (lead institution), the Univ. of Michigan, and Missouri Univ. of S&T in partnerships with over 40 global companies including P&G, Toyota, GE Aviation, Boeing, Caterpillar, Siemens, Harley-Davidson, ITRI (Taiwan), Omron (Japan), Parker Hannifin, Spirit AeroSystems, Nissan (Japan), Syncrude (Canada), CISCO, Alstom (France), Delta Electronics (Taiwan), Ingersoll Rand, Goodyear, Army Research Lab. etc.
He is a honorary professor of City Univ. of Hong Kong and serve as visiting professor for a number of institutions including, Shanghai Jiao Tong Univ., Cranfield Univ. in UK, Lulea Univ. of Technology in Sweden, Hong Kong PolyU., Xian Jiao Tong Univ. and Harbin Institute of Technology (HIT) in China, etc. His current research focuses on dominant innovation design tools and smart infotronics technologies for service innovation applications.
Previously, he served as Director for Product Development and Manufacturing at United Technologies Research Center (UTRC), E. Hartford, CT as well as Program Directors for a number of programs at NSF during 1991-1998, including the Engineering Research Centers (ERCs) Program, the Industry/University Cooperative Research Centers (I/UCRCs) Program, and the Div. of Design, Manufacture, and Industrial Innovation.
Currently, he serves as advisor to a number of global organizations, including IBM MAXIMO Executive Advisory Council, Industrial Technology Research Institute (ITRI) in Taiwan, Japan Productivity Center (JPC), Academy of Machinery Science & Technology in China, etc. In addition, he serves as editors and associate editor for a number of journals including IEEE Transaction on Industrial Informatics, Int. Journal on Prognostics & Health Management (IJPHM), Int. Journal of Asset Engineering an Mgt, Int. Journal on Service Operations and Informatics, Tsinghua Science & Technology Journal, etc, He has authored/co-authored over 150 technical publications, edited two books, contributed numerous book chapters, own a number of patents, 2 trademarks, and had delivered numerous invited lectures and speeches, including over 140 invited keynote and plenary speeches at major international conferences. He is a Fellow of ASME, SME, as well as a founding fellow of International Society of Engineering Asset Management (ISEAM).
David Siegel
PhD Student, IMS Center, University of Cincinnati, Ohio, USA (siegeldn@mail.uc.edu)
David Siegel is currently a PHD student at the University of Cincinnati and a research assistant for the Center for Intelligent Maintenance Systems directed by Professor Jay Lee. He joined the Center in 2007 and has previous experience in over 5 different industrial projects related to the field of prognostics and health management. Previous projects include developing a health monitoring method for a HMMWV vehicle alternator component in a phase I project with TARDEC, developing various health monitoring and signal processing methods for rolling element bearings for a machine tool application, as well as investigation smart sensor technology and algorithms for predicting hydraulic hose failure. Related industrial experience includes a 3 month internship at General Electric Aviation Prognostic and Diagnostic Center to investigate techniques and algorithms for aircraft engine diagnostics and prognostics. David also was part of a two student team that won the Prognostics and Health Management 2009 Data challenge student division and 2nd overall, in which the algorithm scored was judged based on the accuracy of the health information for the gearbox components. David currently has 3 published conference papers as well as 2 journal papers submitted for review. His current research interest includes novel signal processing techniques for gear or bearing related components as well as multi-regime health monitoring and prognostic methods.
|