2016 Western New York Image and Signal Processing
Workshop
The Western New York Image and
Signal Processing Workshop (WNYISPW) is a venue for
promoting image and signal processing research in our area
and for facilitating interaction between academic
researchers, industry researchers, and students. The
workshop comprises both oral and poster presentations.
The workshop, building off of 18 successful years of the Western New York Image Processing Workshop (WNYIPW), is sponsored by the Rochester chapter of the IEEE Signal Processing Society with technical cooperation from the Rochester chapter of the Society for Imaging Science and Technology.
The workshop will be held on Friday, November 18, 2016, in Louise Slaughter Hall (Building SLA/078) at Rochester Institute of Technology in Rochester, NY.
Topics
Topics include, but are not limited to:
Important Dates
Dr. Jiebo Luo, Department of Computer Science at University of Rochester
"Video and Language"
Abstract:
Video has become ubiquitous on the Internet, TV, as well as personal devices. Recognition of video content has been a fundamental challenge in computer vision for decades, where previous research predominantly focused on understanding videos using a predefined yet limited vocabulary. Thanks to the recent development of deep learning techniques, researchers in both computer vision and multimedia communities are now striving to bridge videos with natural language, which can be regarded as the ultimate goal of video understanding. We will present recent advances in exploring the synergy of video understanding and language processing techniques, including video-language alignment, video captioning, and video emotion analysis.
Bio:
Professor Jiebo Luo joined the University of Rochester (UR) in 2011 after a prolific career of over fifteen years at Kodak Research Laboratories. His research spans computer vision, machine learning, data mining, social media, biomedical informatics, and ubiquitous computing. He has published extensively in these fields with 270+ peer-reviewed papers and 90+ granted US patents. He has been involved in numerous technical conferences, including serving as the program chair of ACM Multimedia 2010, IEEE CVPR 2012, and IEEE ICIP 2017. He has served on the editorial boards of the IEEE Transactions on Pattern Analysis and Machine Intelligence (TPAMI), IEEE Transactions on Multimedia (TMM), IEEE Transactions on Circuits and Systems for Video Technology (TCSVT), Pattern Recognition, ACM Transactions on Intelligent Systems and Technology (TIST), Machine Vision and Applications, and Journal of Electronic Imaging. He is a Fellow of the SPIE, IEEE, and IAPR. He is a Data Science CoE Distinguished Researcher with the Georgen Institute for Data Science at UR.
Dr. Jason Yosinski, Geometric Intelligence
"A Deeper Understanding of Large Neural Networks"
Abstract:
Deep neural networks have recently been making a bit of a splash, enabling machines to learn to solve problems that had previously been easy for humans but hard for machines, like playing Atari games or identifying lions or jaguars in photos. But how do these neural nets actually work? What do they learn? This turns out to be a surprisingly tricky question to answer — surprising because we built the networks, but tricky because they are so large and have many millions of connections that effect complex computation which is hard to interpret. Trickiness notwithstanding, in this talk we’ll see what we can learn about neural nets by looking at a few examples of networks in action and experiments designed to elucidate network behavior. The combined experiments yield a better understanding of network behavior and capabilities and promise to bolster our ability to apply neural nets as components in real world computer vision systems.
Bio:
Jason Yosinski is a researcher at Geometric Intelligence, where he uses neural networks and machine learning to build better AI. He was previously a PhD student and NASA Space Technology Research Fellow working at the Cornell Creative Machines Lab, the University of Montreal, the Caltech Jet Propulsion Laboratory, and Google DeepMind.
His work on AI has been featured on NPR, Fast Company, the Economist, TEDx, and on the BBC. When not doing research, Mr. Yosinski enjoys tricking middle school students into learning math while they play with robots.
Invited Industry Partners
Allison Gray, NVIDIA Solutions Architect
"Deep Learning with GPUs"
Abstract:
Deep neural networks have recently been making a bit of a splash, enabling machines to learn to solve problems that had previously been easy for humans but hard for machines, like playing Atari games or identifying lions or jaguars in photos. But how do these neural nets actually work? What do they learn? This turns out to be a surprisingly tricky question to answer — surprising because we built the networks, but tricky because they are so large and have many millions of connections that effect complex computation which is hard to interpret. Trickiness notwithstanding, in this talk we’ll see what we can learn about neural nets by looking at a few examples of networks in action and experiments designed to elucidate network behavior. The combined experiments yield a better understanding of network behavior and capabilities and promise to bolster our ability to apply neural nets as components in real world computer vision systems.
Bio:
Allison Gray is a Solutions Architect at NVIDIA and supports customers interested in using graphics processing units to help them accelerate their applications. Before coming to NVIDIA she was a research engineer at the National Renewable Energy Laboratory, in the Concentrating Solar Power group. She performed surface characterization testing on large aperture solar concentrators. She earned her B.S. and M.S. in Mechanical Engineering from University of Nevada, Las Vegas specializing in thermal sciences. She earned her M.S. in Image Science from RIT, specializing in deep learning.
Johanna Pingel, MathWorks Field Engineer
Mehernaz Savai, MathWorks Field Engineer
Ken Cleveland, MathWorks Senior Account Manager
"Deep Learning and Machine Learning for Image Processing & Computer Vision"
Abstract:
Deep learning applications has rapidly evolved over the past decade and is now being used in fields varying from autonomous systems to medical image processing. This tutorial will cover both Machine learning and Deep learning techniques to help solve problems such as object detection, object recognition and classification. This session will cover demonstrations on 1. Machine Learning Techniques for Scene Classification;
2. Transfer Learning; and
3. Using a pre-trained CNN as a feature extractor.
*All of the code used in the examples will be freely available to all attendees.
Barton Fiske, NVIDIA Business Development Manager
"The NVIDIA Vision"
Abstract:
This presentation will give an overview of where NVIDIA is as a company, helping the audience understand the breadth of its research and application space, and sharing NVIDIA's vision for the future.
Bio:
Barton Fiske is the higher education and research business development manager for NVIDIA focusing on deep learning, HPC and visualization covering the eastern half of the US and Canada. He holds a BSCS in Computer Science from the Rochester Institute of Technology and served as assistant graphics researcher at Brown University in the late 1980s. Prior to joining NVIDIA in May of 2015, Barton has served in a wide variety of roles in the broader IT industry including software developer, systems engineer, technical marketeer, 3d stereo evangelist and product manager in 20+ countries worldwide. Barton is also a co-author and contributing author of books about Java programming for the web.
Invited Speakers
Dr. Zhiyao Duan, Assistant Professor, University of Rochester
"Towards Complete Music Notation Transcription of Piano"
Abstract:
Automatic Music Transcription (AMT), i.e., converting music audio into music notation, is a fundamental problem in music information retrieval and has many potential applications. Existing research on AMT, however, only transcribes music audio into a parametric description such as a MIDI piano-roll. This representation does not carry music knowledge as music notation does, and is not intuitive for humans to interpret. There are two obstacles in designing AMT systems that convert music audio all the way up to music notation: 1) the low accuracy in parametric transcription and 2) the lack of musical knowledge in the transcription process. In this talk, I will present our recent work on addressing these challenges. We employ convolutional sparse coding to obtain an accurate parametric transcription of piano in a context-dependent way. We then convert the piano-roll into music notation by incorporating musical knowledge into the transcription process. Experiments show that our method outperforms state-of-the-art approaches at both the parametric transcription level and the music notation level.
Bio:
Zhiyao Duan is an assistant professor and director of the Audio Information Research (AIR) lab in the Department of Electrical and Computer Engineering at the University of Rochester. He received his B.S. in Automation and M.S. in Control Science and Engineering from Tsinghua University, China, in 2004 and 2008, respectively, and received his Ph.D. in Computer Science from Northwestern University in 2013. His research interest is in the broad area of computer audition, i.e., designing computational systems that are capable of understanding sounds, including music, speech, and environmental sounds. Specific problems that he has been working on include automatic music transcription, audio-score alignment, source separation, speech enhancement, sound retrieval, and audio-visual analysis of music. He co-presented a tutorial on automatic music transcription at the ISMIR conference in 2015.
Dr. Nathan Cahill, Associate Professor, Rochester Institute of Technology
"Data Representations for Exploring Brain Networks"
Abstract:
Graph-based data representation techniques are a staple of brain network analysis. Weighted graphs are constructed from fMRI and/or sMRI data (via correlation of time series from fMRI, or via probabilistic tractography from sMRI), and various quantities derived from these graphs provide mechanisms for exploring the architecure of brain networks of normal and diseased subjects, at both the individual level and groups of subjects. Spectral clustering, which uses eigenvectors of the graph Laplacian matrix to generate a low-dimensional data representation that is amenable to clustering, is a key tool in characterizing both functional and structural network connectivity in the brain. In this talk, we will discuss some recent advances in the underlying data representation techniques upon which spectral clustering is based, and how these advances might be applicable to exploring brain networks
Bio:
Nathan Cahill is an Associate Professor in the School of Mathematical Sciences and an Associate Dean of the College of Science at Rochester Institute of Technology. He directs the Image Computing and Analysis Lab at RIT, which focuses on the development of mathematical models and computational algorithms for the analysis of color, hyperspectral, and medical imagery in a variety of applications. He has published over 60 journal and conference papers to date and is an inventor on 26 US patents. He is a Senior Member of IEEE.
Dr. Christopher Kanan, Assistant Professor, Rochester Institute of Technology
"Visual Question Answering: Algorithms, Datasets, & Challenges"
Abstract:
Algorithms for extracting semantic information from images and video have dramatically improved over the past four years, with today’s best deep convolutional neural networks (CNNs) now rivaling humans at image recognition. These successes have prompted researchers to pursue building new systems that are capable of a multitude of tasks. In Visual Question Answering (VQA), an algorithm is given a text-based question about an image, and it must produce an answer. Although the first VQA datasets were released less than two years ago, algorithms are already approaching human performance. However, these results may be misleading due to biases in existing benchmarks. In this talk, I review the current state of VQA algorithms, including algorithms from my lab. I then analyze existing datasets for VQA and demonstrate that they have severe flaws and limitations. Lastly, I discuss what a better dataset would look like, and examine which kinds of questions are easy and which are hard.
Bio:
Christopher Kanan is an assistant professor in the Chester F. Carlson Center for Imaging Science at the Rochester Institute of Technology. His lab uses machine learning, especially deep learning, to solve problems in computer vision, with an emphasis on task-driven algorithms for understanding images and videos. He is also working on incorporating brain-inspired mechanisms into neural networks. Dr. Kanan received a Ph.D. in computer science from the University of California at San Diego, and an M.S. in computer science from the University of Southern California. Before coming to RIT, Dr. Kanan was a postdoctoral scholar at the California Institute of Technology, and later worked as a Research Technologist at NASA’s Jet Propulsion Laboratory, where he used deep learning to develop vision systems for autonomous ships.
Neeti Narayan, PhD student, SUNY Buffalo
"Automated Analysis of People in Unconstrained Scenarios"
Abstract:
Automated analysis of large amounts of video data can not only process the data faster but significantly improve the quality of surveillance. Video analysis can enable long term activity and behavior characterization of people in a scene. Such analysis is required for high-level surveillance tasks like anomaly detection or undesirable event prediction for timely alerts making surveillance more pro-active. Camera network tracking and object re-identification are major challenges in machine vision based video surveillance. Person re-identification is the problem of automatically searching an individual's presence in a surveillance video. Much of the research on person re-identification has concentrated on ranking individuals in a gallery of known target images given one or more images of an unknown target. In real-time, it is necessary to make continuous entity associations. We develop a framework for continuous re-identification and tracking by exploiting biometric features and other metadata from each individual. We intend to do smoothing for re-identification by treating the observations as noisy outputs of a true hidden state and run the Viterbi algorithm to find the most likely trajectory of the hidden states. This would motivate a coarse-ish discretization of space.
Bio:
Neeti Narayan is a Ph.D. candidate in Center for Unified Biometrics and Sensors (CUBS) at University at Buffalo (UB). She completed her Master's program at UB in 2014 with a focus on machine learning and data mining. Her current research interests are in person tracking and re-identification, video analysis to enable long term activity and behavior characterization of people. Previously she has worked on facial keypoints detection using deep learning, face alignment in unconstrained imagery and liveness detection to overcome face spoofing attacks. In the past, she have worked at Ericsson as a software engineer intern.
Shagan Sah, PhD student, Rochester Institute of Technology
"Video Redaction"
Abstract:
With increasing vast collections of surveillance, body worn camera and private videos, video redaction technology has become very important. Video redaction is obfuscation of personal information in videos for privacy protection. Two primary steps in a visual redaction system are localization of object(s) to be redacted and to obfuscate it. Existing techniques have a manual object tagging step and also require a skilled technician to manually review the accuracy of the system. The process can be expedited by incorporating automated redaction mechanisms which would obfuscate sensitive and privacy revealing information. These mechanisms rely on robust tracking methods across the video to ensure the redaction of all sensitive information while minimizing spurious detections. To demonstrate similar applications, we apply state of the art object detection and tracking algorithms on surveillance videos. Recent studies have explored the use of convolution neural networks and recurrent neural networks for object detection and tracking. We use these models for video redaction and show improvements when detecting human heads compared with faces.
Bio:
Shagan Sah obtained a Bachelors in Engineering degree from the University of Pune, India in 2009. This was followed by a Master of Science degree in Imaging Science from Rochester Institute of Technology (RIT), New York, USA in 2012 with aid of RIT Graduate Scholarship. He is currently a Ph.D. candidate in the Center for Imaging Science at RIT. He is interested in the intersection of machine learning, natural language and computer vision. His current work primarily lies in the applications of deep learning for image and video understanding. In the past, he has worked at Xerox-PARC as a Video Analytics Intern and at Cisco Systems as a Software Engineer. He also had a stint with the National Disaster Management Authority, Government of India as a Senior Research Officer.
Paper Submission
The Call for Papers can be found here.
Paper submission deadline has been extended
to 11:59pm EDT on October 31, 2016.
Prospective authors are invited to submit a 4-page paper + 5th page of references here: https://cmt3.research.microsoft.com/WNYISPW2016/
Authors should use the same formatting/templates described in the ICIP 2015 Paper Kit.
All accepted papers are expected to be included in IEEE Xplore and will be indexed by EI. Past WNYIPW and WNYISPW proceedings can be found here:
Prospective authors are invited to submit an abstract here: https://cmt3.research.microsoft.com/WNYISPW2016/
Awards
To encourage student participation, a best student paper and best student poster
award will be given.
Parking Instructions
Any non-RIT attendees are allowed to park in either Lot T or
the Global Village Lot and then walk to Louise Slaughter
Hall (SLA Building). See the
campus map with parking information (you need to print out a parking pass and
place on your windshield). If you forget to print out a permit,
Non-RIT visitors can stop by the RIT Welcome Center (flagpole entrance) on the
day of the Workshop to get a parking pass.
Detailed Schedule (Tentative)
Organizing Committee
The workshop, building off of 18 successful years of the Western New York Image Processing Workshop (WNYIPW), is sponsored by the Rochester chapter of the IEEE Signal Processing Society with technical cooperation from the Rochester chapter of the Society for Imaging Science and Technology.
The workshop will be held on Friday, November 18, 2016, in Louise Slaughter Hall (Building SLA/078) at Rochester Institute of Technology in Rochester, NY.
Topics
Topics include, but are not limited to:- Formation, Processing, and/or Analysis of Signals, Images, or Video
- Computer Vision
- Information Retrieval
- Image and Color Science
- Applications of Image and Signal Processing, including:
- Medical Image and Signal Analysis
- Audio Processing and Analysis
- Remote Sensing
- Archival Imaging
- Printing
- Consumer Devices
- Security
- Surveillance
- Document Imaging
- Art Restoration and Analysis
- Astronomy
Important Dates
Paper submission opens: | September 23, 2016 |
Paper submission closes: |
October 31, 2016 |
Notification of Acceptance: | November 7, 2016 |
Early (online) registration deadline: | November 9, 2016 |
Submission of camera-ready paper: | November 11, 2016 |
Workshop: | November 18, 2016 |
Keynote Speakers
We are happy to announce that our keynote speakers will be:Dr. Jiebo Luo, Department of Computer Science at University of Rochester
"Video and Language"
Abstract:
Video has become ubiquitous on the Internet, TV, as well as personal devices. Recognition of video content has been a fundamental challenge in computer vision for decades, where previous research predominantly focused on understanding videos using a predefined yet limited vocabulary. Thanks to the recent development of deep learning techniques, researchers in both computer vision and multimedia communities are now striving to bridge videos with natural language, which can be regarded as the ultimate goal of video understanding. We will present recent advances in exploring the synergy of video understanding and language processing techniques, including video-language alignment, video captioning, and video emotion analysis.
Bio:
Professor Jiebo Luo joined the University of Rochester (UR) in 2011 after a prolific career of over fifteen years at Kodak Research Laboratories. His research spans computer vision, machine learning, data mining, social media, biomedical informatics, and ubiquitous computing. He has published extensively in these fields with 270+ peer-reviewed papers and 90+ granted US patents. He has been involved in numerous technical conferences, including serving as the program chair of ACM Multimedia 2010, IEEE CVPR 2012, and IEEE ICIP 2017. He has served on the editorial boards of the IEEE Transactions on Pattern Analysis and Machine Intelligence (TPAMI), IEEE Transactions on Multimedia (TMM), IEEE Transactions on Circuits and Systems for Video Technology (TCSVT), Pattern Recognition, ACM Transactions on Intelligent Systems and Technology (TIST), Machine Vision and Applications, and Journal of Electronic Imaging. He is a Fellow of the SPIE, IEEE, and IAPR. He is a Data Science CoE Distinguished Researcher with the Georgen Institute for Data Science at UR.
Dr. Jason Yosinski, Geometric Intelligence
"A Deeper Understanding of Large Neural Networks"
Abstract:
Deep neural networks have recently been making a bit of a splash, enabling machines to learn to solve problems that had previously been easy for humans but hard for machines, like playing Atari games or identifying lions or jaguars in photos. But how do these neural nets actually work? What do they learn? This turns out to be a surprisingly tricky question to answer — surprising because we built the networks, but tricky because they are so large and have many millions of connections that effect complex computation which is hard to interpret. Trickiness notwithstanding, in this talk we’ll see what we can learn about neural nets by looking at a few examples of networks in action and experiments designed to elucidate network behavior. The combined experiments yield a better understanding of network behavior and capabilities and promise to bolster our ability to apply neural nets as components in real world computer vision systems.
Bio:
Jason Yosinski is a researcher at Geometric Intelligence, where he uses neural networks and machine learning to build better AI. He was previously a PhD student and NASA Space Technology Research Fellow working at the Cornell Creative Machines Lab, the University of Montreal, the Caltech Jet Propulsion Laboratory, and Google DeepMind.
His work on AI has been featured on NPR, Fast Company, the Economist, TEDx, and on the BBC. When not doing research, Mr. Yosinski enjoys tricking middle school students into learning math while they play with robots.
Invited Industry Partners
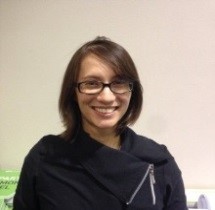
"Deep Learning with GPUs"
Abstract:
Deep neural networks have recently been making a bit of a splash, enabling machines to learn to solve problems that had previously been easy for humans but hard for machines, like playing Atari games or identifying lions or jaguars in photos. But how do these neural nets actually work? What do they learn? This turns out to be a surprisingly tricky question to answer — surprising because we built the networks, but tricky because they are so large and have many millions of connections that effect complex computation which is hard to interpret. Trickiness notwithstanding, in this talk we’ll see what we can learn about neural nets by looking at a few examples of networks in action and experiments designed to elucidate network behavior. The combined experiments yield a better understanding of network behavior and capabilities and promise to bolster our ability to apply neural nets as components in real world computer vision systems.
Bio:
Allison Gray is a Solutions Architect at NVIDIA and supports customers interested in using graphics processing units to help them accelerate their applications. Before coming to NVIDIA she was a research engineer at the National Renewable Energy Laboratory, in the Concentrating Solar Power group. She performed surface characterization testing on large aperture solar concentrators. She earned her B.S. and M.S. in Mechanical Engineering from University of Nevada, Las Vegas specializing in thermal sciences. She earned her M.S. in Image Science from RIT, specializing in deep learning.
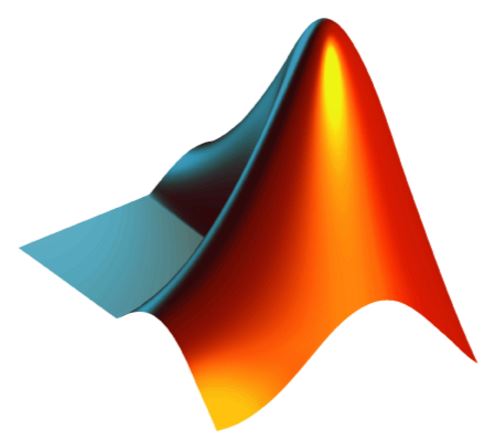
Mehernaz Savai, MathWorks Field Engineer
Ken Cleveland, MathWorks Senior Account Manager
"Deep Learning and Machine Learning for Image Processing & Computer Vision"
Abstract:
Deep learning applications has rapidly evolved over the past decade and is now being used in fields varying from autonomous systems to medical image processing. This tutorial will cover both Machine learning and Deep learning techniques to help solve problems such as object detection, object recognition and classification. This session will cover demonstrations on 1. Machine Learning Techniques for Scene Classification;
2. Transfer Learning; and
3. Using a pre-trained CNN as a feature extractor.
*All of the code used in the examples will be freely available to all attendees.
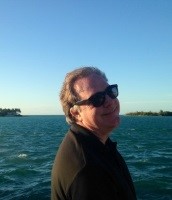
"The NVIDIA Vision"
Abstract:
This presentation will give an overview of where NVIDIA is as a company, helping the audience understand the breadth of its research and application space, and sharing NVIDIA's vision for the future.
Bio:
Barton Fiske is the higher education and research business development manager for NVIDIA focusing on deep learning, HPC and visualization covering the eastern half of the US and Canada. He holds a BSCS in Computer Science from the Rochester Institute of Technology and served as assistant graphics researcher at Brown University in the late 1980s. Prior to joining NVIDIA in May of 2015, Barton has served in a wide variety of roles in the broader IT industry including software developer, systems engineer, technical marketeer, 3d stereo evangelist and product manager in 20+ countries worldwide. Barton is also a co-author and contributing author of books about Java programming for the web.
Invited Speakers
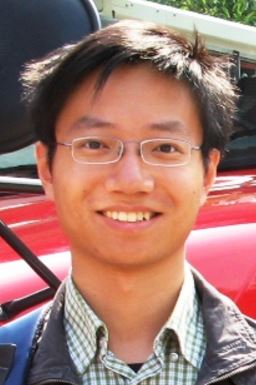
"Towards Complete Music Notation Transcription of Piano"
Abstract:
Automatic Music Transcription (AMT), i.e., converting music audio into music notation, is a fundamental problem in music information retrieval and has many potential applications. Existing research on AMT, however, only transcribes music audio into a parametric description such as a MIDI piano-roll. This representation does not carry music knowledge as music notation does, and is not intuitive for humans to interpret. There are two obstacles in designing AMT systems that convert music audio all the way up to music notation: 1) the low accuracy in parametric transcription and 2) the lack of musical knowledge in the transcription process. In this talk, I will present our recent work on addressing these challenges. We employ convolutional sparse coding to obtain an accurate parametric transcription of piano in a context-dependent way. We then convert the piano-roll into music notation by incorporating musical knowledge into the transcription process. Experiments show that our method outperforms state-of-the-art approaches at both the parametric transcription level and the music notation level.
Bio:
Zhiyao Duan is an assistant professor and director of the Audio Information Research (AIR) lab in the Department of Electrical and Computer Engineering at the University of Rochester. He received his B.S. in Automation and M.S. in Control Science and Engineering from Tsinghua University, China, in 2004 and 2008, respectively, and received his Ph.D. in Computer Science from Northwestern University in 2013. His research interest is in the broad area of computer audition, i.e., designing computational systems that are capable of understanding sounds, including music, speech, and environmental sounds. Specific problems that he has been working on include automatic music transcription, audio-score alignment, source separation, speech enhancement, sound retrieval, and audio-visual analysis of music. He co-presented a tutorial on automatic music transcription at the ISMIR conference in 2015.
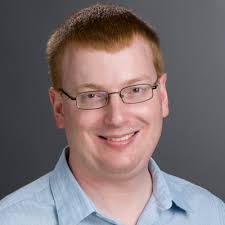
"Data Representations for Exploring Brain Networks"
Abstract:
Graph-based data representation techniques are a staple of brain network analysis. Weighted graphs are constructed from fMRI and/or sMRI data (via correlation of time series from fMRI, or via probabilistic tractography from sMRI), and various quantities derived from these graphs provide mechanisms for exploring the architecure of brain networks of normal and diseased subjects, at both the individual level and groups of subjects. Spectral clustering, which uses eigenvectors of the graph Laplacian matrix to generate a low-dimensional data representation that is amenable to clustering, is a key tool in characterizing both functional and structural network connectivity in the brain. In this talk, we will discuss some recent advances in the underlying data representation techniques upon which spectral clustering is based, and how these advances might be applicable to exploring brain networks
Bio:
Nathan Cahill is an Associate Professor in the School of Mathematical Sciences and an Associate Dean of the College of Science at Rochester Institute of Technology. He directs the Image Computing and Analysis Lab at RIT, which focuses on the development of mathematical models and computational algorithms for the analysis of color, hyperspectral, and medical imagery in a variety of applications. He has published over 60 journal and conference papers to date and is an inventor on 26 US patents. He is a Senior Member of IEEE.
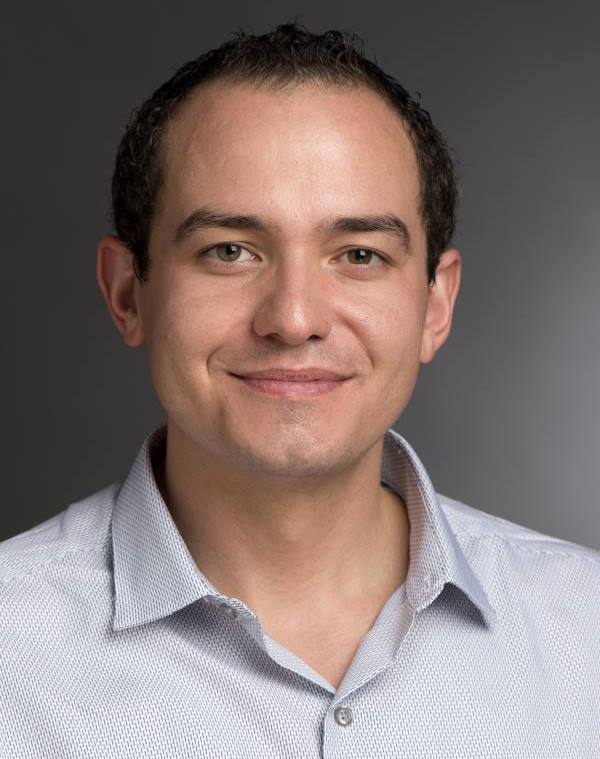
"Visual Question Answering: Algorithms, Datasets, & Challenges"
Abstract:
Algorithms for extracting semantic information from images and video have dramatically improved over the past four years, with today’s best deep convolutional neural networks (CNNs) now rivaling humans at image recognition. These successes have prompted researchers to pursue building new systems that are capable of a multitude of tasks. In Visual Question Answering (VQA), an algorithm is given a text-based question about an image, and it must produce an answer. Although the first VQA datasets were released less than two years ago, algorithms are already approaching human performance. However, these results may be misleading due to biases in existing benchmarks. In this talk, I review the current state of VQA algorithms, including algorithms from my lab. I then analyze existing datasets for VQA and demonstrate that they have severe flaws and limitations. Lastly, I discuss what a better dataset would look like, and examine which kinds of questions are easy and which are hard.
Bio:
Christopher Kanan is an assistant professor in the Chester F. Carlson Center for Imaging Science at the Rochester Institute of Technology. His lab uses machine learning, especially deep learning, to solve problems in computer vision, with an emphasis on task-driven algorithms for understanding images and videos. He is also working on incorporating brain-inspired mechanisms into neural networks. Dr. Kanan received a Ph.D. in computer science from the University of California at San Diego, and an M.S. in computer science from the University of Southern California. Before coming to RIT, Dr. Kanan was a postdoctoral scholar at the California Institute of Technology, and later worked as a Research Technologist at NASA’s Jet Propulsion Laboratory, where he used deep learning to develop vision systems for autonomous ships.
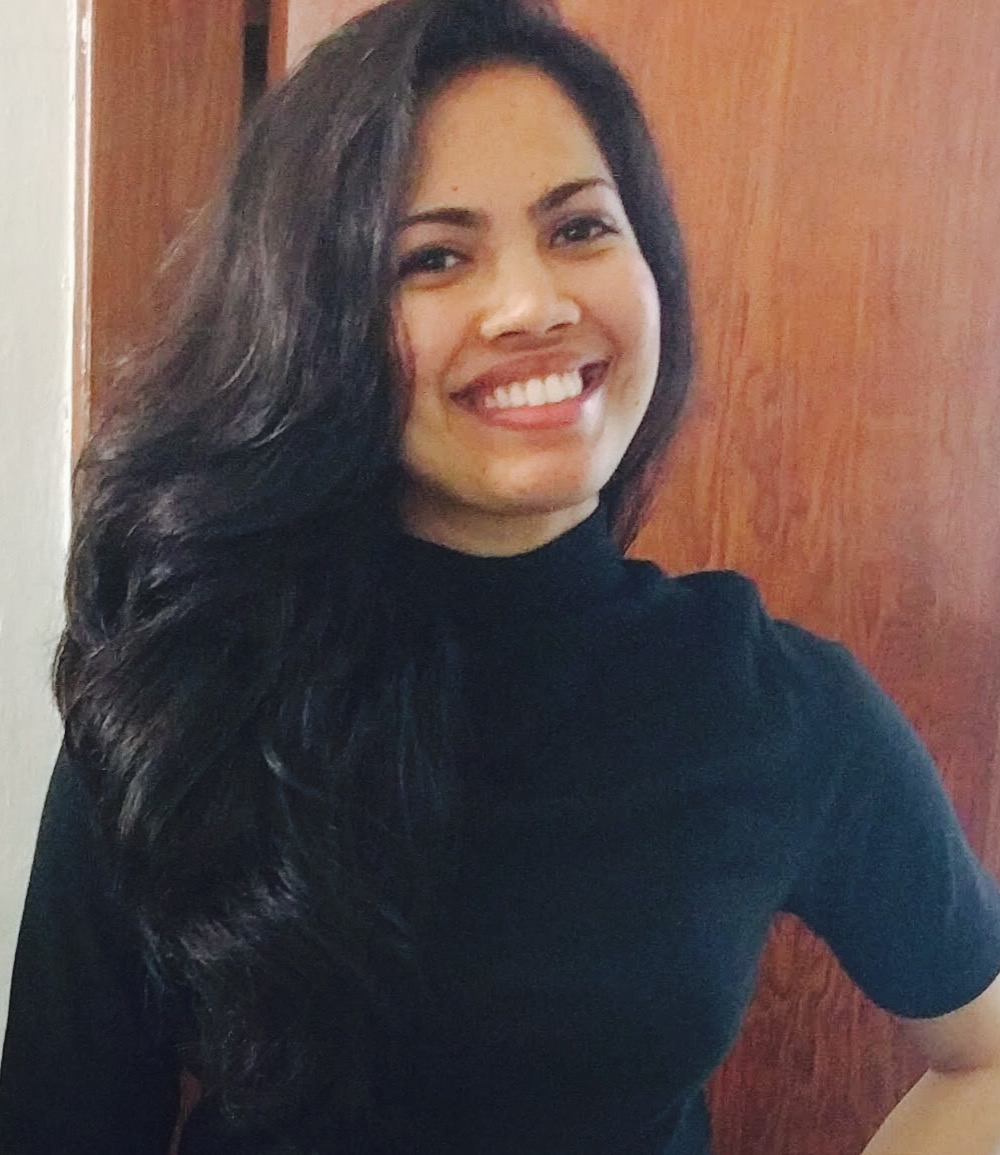
"Automated Analysis of People in Unconstrained Scenarios"
Abstract:
Automated analysis of large amounts of video data can not only process the data faster but significantly improve the quality of surveillance. Video analysis can enable long term activity and behavior characterization of people in a scene. Such analysis is required for high-level surveillance tasks like anomaly detection or undesirable event prediction for timely alerts making surveillance more pro-active. Camera network tracking and object re-identification are major challenges in machine vision based video surveillance. Person re-identification is the problem of automatically searching an individual's presence in a surveillance video. Much of the research on person re-identification has concentrated on ranking individuals in a gallery of known target images given one or more images of an unknown target. In real-time, it is necessary to make continuous entity associations. We develop a framework for continuous re-identification and tracking by exploiting biometric features and other metadata from each individual. We intend to do smoothing for re-identification by treating the observations as noisy outputs of a true hidden state and run the Viterbi algorithm to find the most likely trajectory of the hidden states. This would motivate a coarse-ish discretization of space.
Bio:
Neeti Narayan is a Ph.D. candidate in Center for Unified Biometrics and Sensors (CUBS) at University at Buffalo (UB). She completed her Master's program at UB in 2014 with a focus on machine learning and data mining. Her current research interests are in person tracking and re-identification, video analysis to enable long term activity and behavior characterization of people. Previously she has worked on facial keypoints detection using deep learning, face alignment in unconstrained imagery and liveness detection to overcome face spoofing attacks. In the past, she have worked at Ericsson as a software engineer intern.
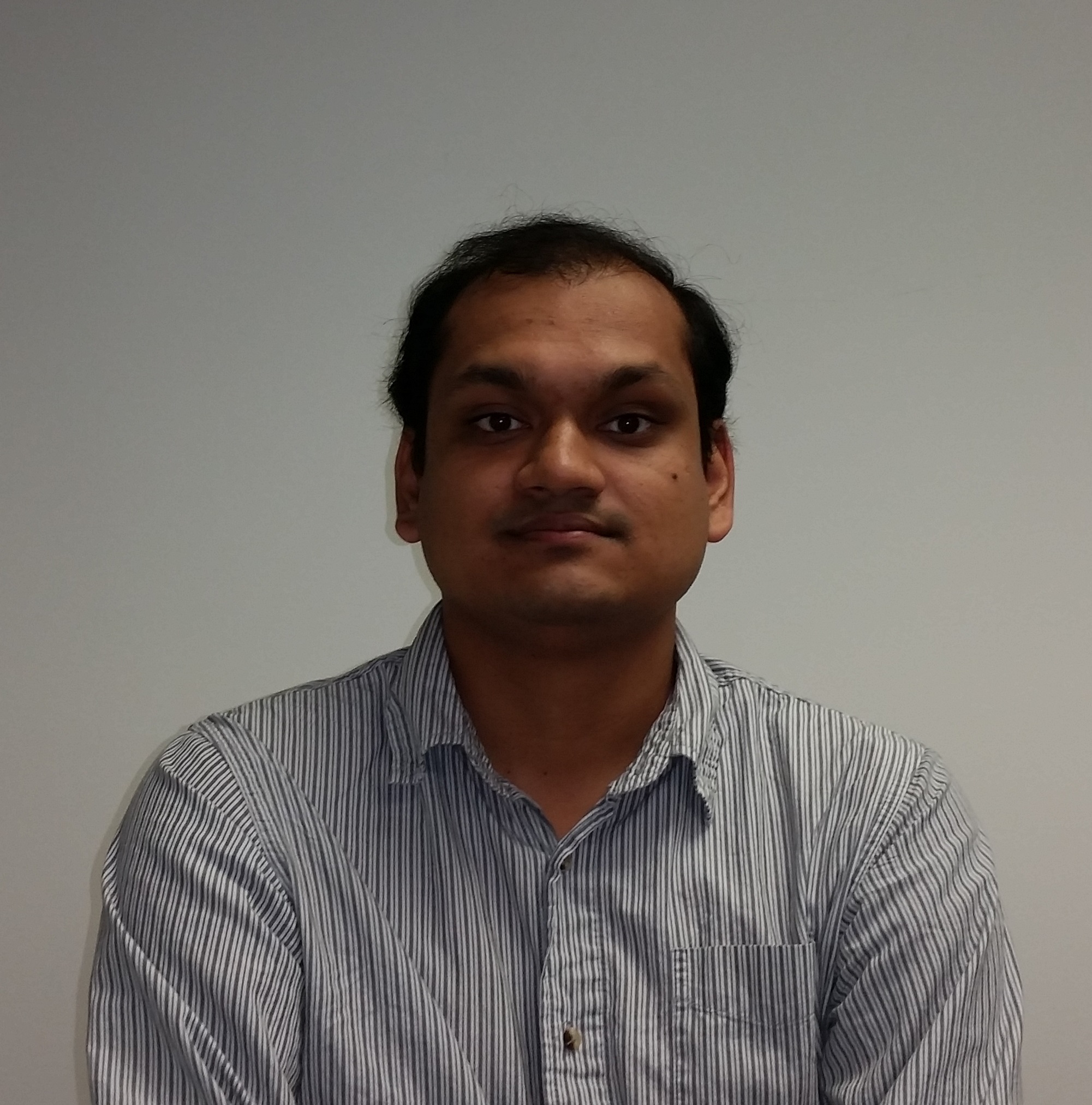
"Video Redaction"
Abstract:
With increasing vast collections of surveillance, body worn camera and private videos, video redaction technology has become very important. Video redaction is obfuscation of personal information in videos for privacy protection. Two primary steps in a visual redaction system are localization of object(s) to be redacted and to obfuscate it. Existing techniques have a manual object tagging step and also require a skilled technician to manually review the accuracy of the system. The process can be expedited by incorporating automated redaction mechanisms which would obfuscate sensitive and privacy revealing information. These mechanisms rely on robust tracking methods across the video to ensure the redaction of all sensitive information while minimizing spurious detections. To demonstrate similar applications, we apply state of the art object detection and tracking algorithms on surveillance videos. Recent studies have explored the use of convolution neural networks and recurrent neural networks for object detection and tracking. We use these models for video redaction and show improvements when detecting human heads compared with faces.
Bio:
Shagan Sah obtained a Bachelors in Engineering degree from the University of Pune, India in 2009. This was followed by a Master of Science degree in Imaging Science from Rochester Institute of Technology (RIT), New York, USA in 2012 with aid of RIT Graduate Scholarship. He is currently a Ph.D. candidate in the Center for Imaging Science at RIT. He is interested in the intersection of machine learning, natural language and computer vision. His current work primarily lies in the applications of deep learning for image and video understanding. In the past, he has worked at Xerox-PARC as a Video Analytics Intern and at Cisco Systems as a Software Engineer. He also had a stint with the National Disaster Management Authority, Government of India as a Senior Research Officer.
Paper Submission
The Call for Papers can be found here.
Paper submission deadline has been extended
to 11:59pm EDT on October 31, 2016. Prospective authors are invited to submit a 4-page paper + 5th page of references here: https://cmt3.research.microsoft.com/WNYISPW2016/
Authors should use the same formatting/templates described in the ICIP 2015 Paper Kit.
All accepted papers are expected to be included in IEEE Xplore and will be indexed by EI. Past WNYIPW and WNYISPW proceedings can be found here:
Poster Submission
Authors who only want to be considered for a poster presentaiton have the option to submit an abstract in place of a full paper. Poster presentors will be asked to give a 60 second lightning round presentation at the conference. (Note: Abstract-only submissions will not be searchable on IEEE Xplore)Prospective authors are invited to submit an abstract here: https://cmt3.research.microsoft.com/WNYISPW2016/
Author Attendance
At least one author of each accepted paper or poster must register and attend the workshop to give an oral or poster presentation. Failure to present the paper will result in automatic withdrawal of the paper from being published in the proceedings.Awards
To encourage student participation, a best student paper and best student poster
award will be given. Registration
Registration is available online here. Onsite registration will be also available, with onsite registration fees payable by cash or check. Fees enable attendance to all sessions and include breakfast, lunch, and afternoon snack. Registration fees are:- General Registration: $50 (with online registration by
11/9), $60 (online after 11/9 or onsite)
- Student Registration: $30 (with online registration by 11/9), $40 (online after 11/9 or onsite)
- IEEE or IS&T Membership: $30 (with online registration by 11/9), $40 (online after 11/9 or onsite)
- IEEE or IS&T Student Membership: $20 (with online registration by 11/9), $30 (online after 11/9 or onsite)
Conference at a Glance (Detailed Schedule below)
- 8:15-8:50am, Registration, breakfast
- 8:50-9am, Welcome
- 9-11am, Oral presentations
- 9-11am, Deep learning tutorial by MathWorks
- 11am-Noon, Keynote: Jason Yosinski
- Noon-12:30, Poster spotlights
- 12:30-2pm, Lunch and posters
- 2-3pm, Keynote: Jiebo Luo
- 3-5pm, Oral presentations
- 3-5pm, Deep learning tutorial by NVIDIA
- 5-5:15pm, Awards
Oral Presentation Instructions
All oral presentations will be 12 minutes long plus 2 minutes for questions. Presentors supply their own laptop with a VGA connector. (Note: there are no HDMI connectors.) Morning/afternoon presentors should test their laptop on the display screen during the 8:15-8:45am or 12:30-1:45pm timeframes respectively. Papers whose first author is a student qualify for best paper award.Poster Presentation Instructions
All printed posters must be no wider than 40" wide x 48" tall. Poster stations will be available for both mounted and unmounted posters. (If you can bring a mounted poster, please do so.) Attachment materials will be provided. All posters must be displayed by 11am and removed by 5:30pm. There are no electrical outlets next to the poster displays. All poster presentors qualify for a 60 second poster spotlight in the noon-12:30pm timeframe. If you would like to participate in this, please send a one page PDF slide to the conference organizer by November 16th. Posters whose first author is a student qualify for best poster award.Parking Instructions
Any non-RIT attendees are allowed to park in either Lot T or
the Global Village Lot and then walk to Louise Slaughter
Hall (SLA Building). See the
campus map with parking information (you need to print out a parking pass and
place on your windshield). If you forget to print out a permit,
Non-RIT visitors can stop by the RIT Welcome Center (flagpole entrance) on the
day of the Workshop to get a parking pass.Detailed Schedule (Tentative)
- 8:15-8:50am, Registration, breakfast (Rooms 2210-2240)
- 8:50-9am, Welcome by Conference Chair (Rooms 2210-2240)
- 9-11am, Oral presentations (Rooms 2210-2240)
- 9am: Invited talk: "Towards Complete Music Notation Transcription of Piano", Zhiyao Duan, Assistant Professor, University of Rochester
- 9:20am: Invited talk: "The NVIDIA Vision", Barton Fiske, NVIDIA Business Development Manager
- 9:45am: Invited talk: “Data Representations for Exploring Brain Networks”, Nathan Cahill, Rochester Institute of Technology
- 10:10am: “An Acoustic Lens Based Ultrasound Imaging Camera”, Zchao Han, Bhargava Chinni, Vikram Dogra, and Nalvagund Rao, Rochester Institute of Technology
- 10:25am: Invited talk: "Visual Question Answering: Algorithms, Datasets, & Challenges", Christopher Kanan, Assistant Professor, Rochester Institute of Technology
- 10:45-11am: AM break
- 9-11am, MathWorks Tutorial (Room 2140): “Deep Learning and Machine Learning for Image Processing & Computer Vision”
- Johanna Pingel, MathWorks Field Engineer
- Mehernaz Savai, MathWorks Field Engineer
- Ken Cleveland, MathWorks Senior Account Manager
- 11am-Noon, Keynote (Rooms 2210-2240): “A Deeper Understanding of Large Neural Networks”, Jason Yosinski, Geometric Intelligence
- Noon-12:30, Poster spotlights (Rooms 2210-2240)
- 12:30-2pm, Lunch and poster displays (Rooms 2210-2240)
- “Kalman Filter for Total Harmonics Distortion Reduction in Power Systems”, Liqaa Alhafadhi, Johnson Asumadu, and Amean Alsafi, Western Michigan University
- “Total Harmonic Distortion Reduction Using a New Method of Adaptive Filtering”, Liqaa Alhafadhi, Johnson Asumadu, and Amean Alsafi, Western Michigan University
- “Selection of the Best Despeckle Filter of Ultrasound Images”, Ghada Nady Hussien Abd ElGwad, Yasser M. K. Omar, Arab Academy for Science
- “Towards Automatic Cover Song Detection Using Parallel Deep Convolutional Neural Networks”, Marko Stamenovic, University of Rochester
- “Naïve Bayes Pixel-Level Plant Segmentation”, Arash Abbasi and Noah Fahlgren, Donald Danforth Plant Science Center
- “Associating Players to Sound Sources in Musical Performance Videos”, Bochen Li, University of Rochester
- “Text Extraction from Mobile Camera Captured Images”, Deepak Sharma, Rochester Institute of Technology
- “Dysarthric Speech Recognition using Deep Neural Networks”, Suhas Pillai, Rochester Institute of Technology
- “Video Classification and Captioning with Hierarchical Recurrent Neural Network” , Thang Nguyen, Rochester Institute of Technology
- “Fantasy Sports Points Prediction Using Machine Learning”, Dheeraj Kumar Peri, Rohan Dhamdhere, Rochester Institute of Technology
- “Interpreting the Instantaneous Frequency of Reverberant Audio Signals”, Sarah Smith, Mark Bocko, University of Rochester
- “Byte Visualization Method for Malware Classification”, Zhuojun Ren, Guang Chen, Xiuling Han, Donghua University
- “A Convolutional Neural Network (CNN)-based Framework for Skeleton Structure Evaluation using Multi-view Avatar Model”, Daniel Luong, Corbin Dzimian, Adam Moncure, Gang Hu, SUNY Fredonia
- “One Shot Learning for Acoustic Recognition”, Syed Ahmed, Rochester Institute of Technology
- “Tracking by Detection for Video Redaction using Recurrent Neural Network”, Ameya Shringi, Shagan Sah, Raymond Ptucha, Rochester Institute of Technology
- “Musical Audio Feature Extraction Using Deep Networks”, Madeleine Daigneau, Rochester Institute of Technology
- “FPGA based Convolution Neural Network Acceleration”, Felipe Petroski Such, Luke Boudreau, Rochester Institute of Technology
- “Applications of Video Object Segmentation Using a Graph-based Supervoxel Clustering Framework”, Chi Zhang, Alexander Loui, Rochester Institute of Technology
- "Sentiment Analysis of Visual Stimuli", Titus Thomas, Ray Ptucha, Rochester Institute of Technology
- 2-3pm, Keynote (Rooms 2210-2240): “Video and Language”, Jiebo Luo, Department of Computer Science at University of Rochester
- 3-5pm, Oral presentations (Rooms 2210-2240)
- 3pm: "A Local Linear Fitting Based Matting Aproach for Accurate Depth Upsampling", Yanfu Zhang, Li Ding, and Gaurav Sharma, University of Rochester
- 3:15pm: “Motion Tracking for Gesture Analysis in Sports”, Reneta P. Barneva, Valentin E. Brimkov, Patrick Hung, Kamen Kanev, State University of New York at Fredonia
- 3:30pm: “Unsupervised Change Detection Using Spatial Transformer Networks”, Dan Chianucci and Andreas Savakis, Rochester Institute of Technology
- 3:45pm: “Signal Analysis for Detecting Motor Symptoms in Parkinson’s and Huntingtons’s Disease Using Multiple Body-affixed Sensors: A Pilot Study”, Karthik Dinesh, Mulin Xiong, Jamie Adams, Ray Dorsey, Gaurav Sharma, University of Rochester
- 4:00pm: Invited talk: "Video Redaction", Shagan Sah, PhD candidate, Rochester Institute of Technology
- 4:20pm: Invited talk: "Automated analysis of people in unconstrained scenarios", Neeti Narayan, 2015 WNYISPW Best Paper Winner, SUNY/Buffalo
- 4:40-5pm: PM break (Committee meeting to select best paper and best poster)
- 3-5pm, NVIDIA Tutorial (Room 2140): “Deep Learning with GPUs”
- Allison Gray, NVIDIA Solutions Architect
- Barton Fiske, NVIDIA Business Development Manager
- 5-5:15pm, Closing and Awards (Rooms 2210-2240)
Organizing Committee
- Ziya Arnavut, SUNY Fredonia
- Nathan Cahill, Rochester Institute of Technology
- Zhiyao Duan, University of Rochester
- Christopher Kanan, Rochester Institute of Technology
- Paul Lee, University of Rochester
- Cristian Linte, Rochester Institute of Technology
- Michel Molaire, Molaire Consulting LLC
- David Odgers, Odgers Imaging
- Raymond Ptucha, Rochester Institute of Technology
- Beilei Xu, Xerox Corporation