2017 Western New York Image and Signal Processing
Workshop
The Western New York Image and
Signal Processing Workshop (WNYISPW) is a venue for
promoting image and signal processing research in our area
and for facilitating interaction between academic
researchers, industry researchers, and students. The
workshop comprises both oral and poster presentations.
The workshop, building off of 19 successful years of the Western New York Image Processing Workshop (WNYIPW), is sponsored by the Rochester chapter of the IEEE Signal Processing Society with technical cooperation from the Rochester chapter of the Society for Imaging Science and Technology.
The workshop will be held on Friday, November 17, 2017, in Louise Slaughter Hall (Building SLA/078) at Rochester Institute of Technology in Rochester, NY.
Topics
Topics include, but are not limited to:
Important Dates
Dr. David J. Crandall, Associate Professor, School of Informatics, Computing, and Engineering, Indiana University
"Egocentric Computer Vision, for Fun and for Science"
Abstract:
The typical datasets we use to train and test computer vision algorithms consist of millions of consumer-style photos. But this imagery is significantly different from what humans actually see as they go about our daily lives. Low-cost, light wearable cameras (like GoPro) now make it possible to record people's lives from a first-person, "egocentric" perspective that approximates their actual fields of view. What new applications are possible with these devices? How can computer vision contribute to and benefit from this embodied perspective on the world? What could mining datasets of first-person imagery reveal about ourselves and about the world in general? In this talk, I'll describe recent work investigating these questions, focusing on two lines of work on egocentric imagery as examples. The first is for consumer applications, where our goal is to develop automated classifiers to help organize first-person images across several dimensions. The second is an interdisciplinary project using computer vision with wearable cameras to study parent-child interactions in order to better understand child learning. Despite the different goals, these applications share common themes of robustly recognizing image content in noisy, highly dynamic, unstructured imagery.
Bio:
David Crandall is an Associate Professor in the School of Informatics and Computing at Indiana University Bloomington, where he is a member of the programs in Computer Science, Informatics, Cognitive Science, and Data Science, and of the Center for Complex Networks and Systems Research. He received the Ph.D. in computer science from Cornell University in 2008 and the M.S. and B.S. degrees in computer science and engineering from the Pennsylvania State University in 2001. He was a Postdoctoral Research Associate at Cornell from 2008-2010, and a Senior Research Scientist with Eastman Kodak Company from 2001-2003. He has received an NSF CAREER award, a Google Faculty Research Award, best paper awards or nominations at CVPR, CHI, ICDL, ICCV, and WWW, and an Indiana University Trustees Teaching Award.
Dr. Gao Huang, Postdoctoral Fellow in the Department of Computer Science at Cornell University
"Efficient Training and Inference of Very Deep Convolutional Networks"
Abstract:
Recent years have witnessed astonishing progress in convolutional neural networks (CNN). This fast development was largely due to the availability of training and inference of very deep models, which have even managed to surpass human-level performance on many vision tasks. However, the requirements for real world applications differ from those necessary to win competitions, as the computational efficiency becomes a major concern in practice. In the first part of the talk, I will introduce a stochastic depth network, which can significantly speed up the training process of deep models, making them more robust and generalize better. In the second part I will propose a densely connected network (DenseNet), which is inspired by the insights that we obtained from the stochastic depth network. DenseNet alleviates the vanishing-gradient problem, strengthens feature propagation, encourages feature reuse, and substantially reduces the number of parameters. The third part of the talk will focus on how to inference deep models under limited computational resources. I will introduce a multi-scale dense network (MSDNet) with shortcut classifiers, which facilitate retrieving fast and accurate predictions from intermediate layers, leading to significantly improved efficiency over state-of-the-art convolutional networks.
Bio:
Gao Huang is a postdoc researcher from the Department of Computer Science at Cornell University. He received the Ph.D. degree from the Department of Automation at Tsinghua University in 2015, and he was an intern/visiting scholar at Microsoft Research Asia (MSRA), Washington University in St. Louis and Nanyang Technological University. His research interests lie in machine learning and computer vision, with a focus on deep learning algorithms and network architectures. His paper “Densely Connected Convolutional Networks” won the Best Paper Award at CVPR 2017.
Invited Speakers
Dr. Edgar A. Bernal, United Technologies Research Center
"Deep Discriminative and Generative Models for Temporal Multimodal Data Fusion"
Abstract:
Multimodal data fusion aims at achieving synergistic processing of multiple types of data, often in support of decision-making processes. While a variety of approaches including early and late fusion schemes have been proposed, most existing fusion schemes fail to effectively model dependencies across multimodal data that are inherently temporal in nature, such as video, audio, and motion sensor data. While temporal models for data fusion have been proposed in the past, they have traditionally relied on memoryless or short-term memory frameworks which fail to learn long-term cross-modal dependencies from extended experience where temporal gaps between significant events may be highly variable. In this talk, I will introduce deep discriminative and generative models for temporal data fusion which simultaneously learn the joint representation of multiple input modalities as well as the temporal structure within the data. The effectiveness of the proposed models will be demonstrated on automated decision-making tasks including video- and sensor-based action classification, and audiovisual speech recognition.
Bio:
Edgar A. Bernal is a Principal Scientist at the United Technologies Research Center (UTRC) in East Hartford, CT. Prior to joining UTRC, he was a Senior Research Scientist at the Palo Alto Research Center, A Xerox Company, in Webster, NY. He received MSc and PhD degrees in Electrical Engineering from Purdue University, in West Lafayette, IN. His current research interests include computer vision, video-based action and activity recognition, machine and deep learning, and multimodal fusion. He has served as an adjunct faculty member at the Rochester Institute of Technology, Center for Imaging Science, and is a frequent reviewer for IEEE Transactions on Image Processing, IEEE Transactions on Multimedia, the Journal of Electronic Imaging and the Journal of Imaging Science and Technology.
Dr. Dhireesha Kudithipudi, Professor, Rochester Institute of Technology
"On-Device Intelligence : Are we there yet? "
Abstract:
TBD.
Bio:
Dr. Dhireesha Kudithipudi is a professor and director of the NanoComputing Research Laboratory in the Department of Computer Engineering at Rochester Institute of Technology. Her research interests are in designing novel computing substrates that are extremely energy efficient. Her lab looks at brain as one source of inspiration and has designed several neuro-architectures and systems using emerging devices, that are applied in autonomous systems and security. Her current research efforts are supported by ARL, NSA, Sandia National Labs, NSF, AFRL, and Seagate. She is a recipient of the AFRL Faculty Fellowship twice, Telluride Cognitive Computing Fellowship, University presidential Fellowship, and several other awards. She is equally passionate about outreach and organizes workshops for young kids in Rochester area. She is an associate editor of IEEE Transactions on Neural Networks and an invited speaker in numerous DOE/DOD panels, IEEE/ACM workshops and symposia in neuromorphic computing.
Dr. Anh Nguyen, Assistant Professor, University of Auburn
"Plug & Play Generative Networks: Conditional Iterative Generation of Images in Latent Space"
Abstract:
Generating high-resolution, photo-realistic images has been a long-standing goal in machine learning. Recently, Nguyen et al. (2016) showed one interesting way to synthesize novel images by performing gradient ascent in the latent space of a generator network to maximize the activations of one or multiple neurons in a separate classifier network. In this paper we extend this method by introducing an additional prior on the latent code, improving both sample quality and sample diversity, leading to a state-of-the-art generative model that produces high quality images at higher resolutions (227×227) than previous generative models, and does so for all 1000 ImageNet categories. In addition, we provide a unified probabilistic interpretation of related activation maximization methods and call the general class of models “Plug and Play Generative Networks”. PPGNs are composed of (1) a generator network G that is capable of drawing a wide range of image types and (2) a replaceable “condition” network C that tells the generator what to draw. We demonstrate the generation of images conditioned on a class (when C is an ImageNet or MIT Places classification network) and also conditioned on a caption (when C is an image captioning network). Our method also improves the state of the art of Multifaceted Feature Visualization, which generates the set of synthetic inputs that activate a neuron in order to better understand how deep neural networks operate. Finally, we show that our model performs reasonably well at the task of image inpainting. While image models are used in this paper, the approach is modality-agnostic and can be applied to many types of data.
Bio:
Anh Nguyen has completed his Ph.D. study at the University of Wyoming, working with Jeff Clune and Jason Yosinski. His research focus is Deep Learning, specifically understanding and improving deep neural networks. Anh has also worked as a ML research intern at Apple and Uber AI Labs, and a software engineer building front-end user interfaces and visualizations at Bosch and 188bet.com. His research has won Best Paper Awards at CVPR, GECCO, ICML Visualization workshop and Best Research Video Awards at IJCAI and AAAI. His works have also been repeatedly mentioned in MIT Technology Review, Nature, Scientific American and Deep Learning lectures at various institutions.
Dr. Kevin Parker, May Professor of ECE, Dean Emeritus, University of Rochester
"Imaging the BioMechanical Properties of Tissues"
Abstract:
Significant progress has been made in developing ultrasound and MR techniques that use conventional imaging platforms to sense tissue displacements and calculate the intrinsic biomechanical properties such as the stiffness (Young’s Modulus) and the viscosity. These tissue properties are otherwise hidden yet have high diagnostic value for a wide range of conditions from liver fibrosis to breast cancer. We review the scope of these techniques, including pioneering work done at UR, with an overview of some important clinical applications, and the computational challenges.
Bio:
Kevin J. Parker earned his graduate degrees from MIT and has served at the University of Rochester as Professor, Department Chair, Director of the Rochester Center for Biomedical Ultrasound, and Dean of the School of Engineering and Applied Sciences. His research is in image processing and medical imaging, and he is a fellow of the Institute of Electrical and Electronics Engineers (IEEE), the American Institute of Ultrasound in Medicine (AIUM), the Acoustical Society of America (ASA), and the American Institute for Medical and Biological Engineering (AIMBE). He is an inventor or a founder of a number of enterprises, including the field of elastography and the International Conference series in that area, the Blue Noise Mask, and VirtualScopics, Inc. Professor Parker has over 150 journal publications and dozens of US and international patents..
Tutorials
Tim Mathieu, MathWorks Field Engineer
Louvere Walker-Hannon, MathWorks Field Engineer
"Deep Learning for Image Processing & Computer Vision Seminar"
Abstract:
Deep learning techniques have rapidly evolved over the past decade and they are now being used in fields varying from autonomous systems to medical image processing. This session will cover both Machine learning and Deep learning techniques to help solve problems such as object detection, object recognition and classification. This session will cover the following:
Syed Ahmed, Student and Researcher, Rochester Institute of Technology
"Tensorflow Tutorial"
Abstract:
TensorFlow is a powerful library for doing large-scale numerical computation. One of the tasks at which it excels is implementing and training deep neural networks. In this session we will learn about:
Bio:
Syed Tousif Ahmed is majoring in computer engineering at RIT and works there as a Research Assistant in the National Technical Institute for the Deaf. Syed's interests lie in high performance computing, machine intelligence, digital logic design, and cryptography. Syed worked on several production and startup machine intelligence teams such as NVIDIA, NextDroid LLC, and Ahold USA. He has contributed to several open source frameworks such as TensorFlow, Caffe2 and Torch.
Paper Submission
The Call for Papers can be found here.
Prospective authors are invited to submit a 4-page paper + 5th page of references
here: https://cmt3.research.microsoft.com/WNYISPW2017/
Authors should use the same formatting/templates described in the ICIP 2015 Paper Kit.
All accepted papers will be submitted to IEEE Xplore and EI. Past WNYIPW and WNYISPW proceedings can be found here:
Prospective authors are invited to submit an abstract here: https://cmt3.research.microsoft.com/WNYISPW2017/
Awards
To encourage student participation, a best student paper and best student poster
award will be given.
If your department does not have poster printing capabilities, you can get posters printed at "the Hub Express" in the RIT Student Union, hubxpr@rit.edu, 585-475-3471. Color wide format inkjet is $7/sq.ft. Mounting (up to 30x40) is $4/sq.ft. (Contact the Hub Express if you have larger than 30x40)
Parking Instructions
Any non-RIT attendees are allowed to park in either Lot T or
the Global Village Lot and then walk to Louise Slaughter
Hall (SLA Building). See the
campus map with parking information (you need to print out a parking pass and
place on your windshield). If you forget to print out a permit,
Non-RIT visitors can stop by the RIT Welcome Center (flagpole entrance) on the
day of the Workshop to get a parking pass.
Tim Mathieu, MathWorks Field Engineer
Louvere Walker-Hannon, MathWorks Field Engineer
"Deep Learning for Image Processing & Computer Vision Seminar"
Detailed Schedule
Organizing Committee
The workshop, building off of 19 successful years of the Western New York Image Processing Workshop (WNYIPW), is sponsored by the Rochester chapter of the IEEE Signal Processing Society with technical cooperation from the Rochester chapter of the Society for Imaging Science and Technology.
The workshop will be held on Friday, November 17, 2017, in Louise Slaughter Hall (Building SLA/078) at Rochester Institute of Technology in Rochester, NY.
Topics
Topics include, but are not limited to:- Formation, Processing, and/or Analysis of Signals, Images, or Video
- Computer Vision
- Information Retrieval
- Image and Color Science
- Applications of Image and Signal Processing, including:
- Medical Image and Signal Analysis
- Audio Processing and Analysis
- Remote Sensing
- Archival Imaging
- Printing
- Consumer Devices
- Security
- Surveillance
- Document Imaging
- Art Restoration and Analysis
- Astronomy
Important Dates
Paper submission opens: | September 23, 2017 |
Paper submission closes: | October 25, 2017 |
Notification of Acceptance: | November 1, 2017 |
Early (online) registration deadline: | November 3, 2017 |
Submission of camera-ready paper: | November 10, 2017 |
Workshop: | November 17, 2017 |
Keynote Speakers
We are happy to announce our keynote speakers:Dr. David J. Crandall, Associate Professor, School of Informatics, Computing, and Engineering, Indiana University
"Egocentric Computer Vision, for Fun and for Science"
Abstract:
The typical datasets we use to train and test computer vision algorithms consist of millions of consumer-style photos. But this imagery is significantly different from what humans actually see as they go about our daily lives. Low-cost, light wearable cameras (like GoPro) now make it possible to record people's lives from a first-person, "egocentric" perspective that approximates their actual fields of view. What new applications are possible with these devices? How can computer vision contribute to and benefit from this embodied perspective on the world? What could mining datasets of first-person imagery reveal about ourselves and about the world in general? In this talk, I'll describe recent work investigating these questions, focusing on two lines of work on egocentric imagery as examples. The first is for consumer applications, where our goal is to develop automated classifiers to help organize first-person images across several dimensions. The second is an interdisciplinary project using computer vision with wearable cameras to study parent-child interactions in order to better understand child learning. Despite the different goals, these applications share common themes of robustly recognizing image content in noisy, highly dynamic, unstructured imagery.
Bio:
David Crandall is an Associate Professor in the School of Informatics and Computing at Indiana University Bloomington, where he is a member of the programs in Computer Science, Informatics, Cognitive Science, and Data Science, and of the Center for Complex Networks and Systems Research. He received the Ph.D. in computer science from Cornell University in 2008 and the M.S. and B.S. degrees in computer science and engineering from the Pennsylvania State University in 2001. He was a Postdoctoral Research Associate at Cornell from 2008-2010, and a Senior Research Scientist with Eastman Kodak Company from 2001-2003. He has received an NSF CAREER award, a Google Faculty Research Award, best paper awards or nominations at CVPR, CHI, ICDL, ICCV, and WWW, and an Indiana University Trustees Teaching Award.
Dr. Gao Huang, Postdoctoral Fellow in the Department of Computer Science at Cornell University
"Efficient Training and Inference of Very Deep Convolutional Networks"
Abstract:
Recent years have witnessed astonishing progress in convolutional neural networks (CNN). This fast development was largely due to the availability of training and inference of very deep models, which have even managed to surpass human-level performance on many vision tasks. However, the requirements for real world applications differ from those necessary to win competitions, as the computational efficiency becomes a major concern in practice. In the first part of the talk, I will introduce a stochastic depth network, which can significantly speed up the training process of deep models, making them more robust and generalize better. In the second part I will propose a densely connected network (DenseNet), which is inspired by the insights that we obtained from the stochastic depth network. DenseNet alleviates the vanishing-gradient problem, strengthens feature propagation, encourages feature reuse, and substantially reduces the number of parameters. The third part of the talk will focus on how to inference deep models under limited computational resources. I will introduce a multi-scale dense network (MSDNet) with shortcut classifiers, which facilitate retrieving fast and accurate predictions from intermediate layers, leading to significantly improved efficiency over state-of-the-art convolutional networks.
Bio:
Gao Huang is a postdoc researcher from the Department of Computer Science at Cornell University. He received the Ph.D. degree from the Department of Automation at Tsinghua University in 2015, and he was an intern/visiting scholar at Microsoft Research Asia (MSRA), Washington University in St. Louis and Nanyang Technological University. His research interests lie in machine learning and computer vision, with a focus on deep learning algorithms and network architectures. His paper “Densely Connected Convolutional Networks” won the Best Paper Award at CVPR 2017.
Invited Speakers
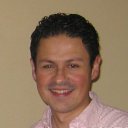
"Deep Discriminative and Generative Models for Temporal Multimodal Data Fusion"
Abstract:
Multimodal data fusion aims at achieving synergistic processing of multiple types of data, often in support of decision-making processes. While a variety of approaches including early and late fusion schemes have been proposed, most existing fusion schemes fail to effectively model dependencies across multimodal data that are inherently temporal in nature, such as video, audio, and motion sensor data. While temporal models for data fusion have been proposed in the past, they have traditionally relied on memoryless or short-term memory frameworks which fail to learn long-term cross-modal dependencies from extended experience where temporal gaps between significant events may be highly variable. In this talk, I will introduce deep discriminative and generative models for temporal data fusion which simultaneously learn the joint representation of multiple input modalities as well as the temporal structure within the data. The effectiveness of the proposed models will be demonstrated on automated decision-making tasks including video- and sensor-based action classification, and audiovisual speech recognition.
Bio:
Edgar A. Bernal is a Principal Scientist at the United Technologies Research Center (UTRC) in East Hartford, CT. Prior to joining UTRC, he was a Senior Research Scientist at the Palo Alto Research Center, A Xerox Company, in Webster, NY. He received MSc and PhD degrees in Electrical Engineering from Purdue University, in West Lafayette, IN. His current research interests include computer vision, video-based action and activity recognition, machine and deep learning, and multimodal fusion. He has served as an adjunct faculty member at the Rochester Institute of Technology, Center for Imaging Science, and is a frequent reviewer for IEEE Transactions on Image Processing, IEEE Transactions on Multimedia, the Journal of Electronic Imaging and the Journal of Imaging Science and Technology.
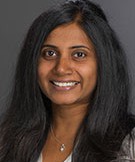
"On-Device Intelligence : Are we there yet? "
Abstract:
TBD.
Bio:
Dr. Dhireesha Kudithipudi is a professor and director of the NanoComputing Research Laboratory in the Department of Computer Engineering at Rochester Institute of Technology. Her research interests are in designing novel computing substrates that are extremely energy efficient. Her lab looks at brain as one source of inspiration and has designed several neuro-architectures and systems using emerging devices, that are applied in autonomous systems and security. Her current research efforts are supported by ARL, NSA, Sandia National Labs, NSF, AFRL, and Seagate. She is a recipient of the AFRL Faculty Fellowship twice, Telluride Cognitive Computing Fellowship, University presidential Fellowship, and several other awards. She is equally passionate about outreach and organizes workshops for young kids in Rochester area. She is an associate editor of IEEE Transactions on Neural Networks and an invited speaker in numerous DOE/DOD panels, IEEE/ACM workshops and symposia in neuromorphic computing.
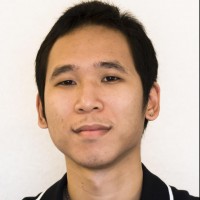
"Plug & Play Generative Networks: Conditional Iterative Generation of Images in Latent Space"
Abstract:
Generating high-resolution, photo-realistic images has been a long-standing goal in machine learning. Recently, Nguyen et al. (2016) showed one interesting way to synthesize novel images by performing gradient ascent in the latent space of a generator network to maximize the activations of one or multiple neurons in a separate classifier network. In this paper we extend this method by introducing an additional prior on the latent code, improving both sample quality and sample diversity, leading to a state-of-the-art generative model that produces high quality images at higher resolutions (227×227) than previous generative models, and does so for all 1000 ImageNet categories. In addition, we provide a unified probabilistic interpretation of related activation maximization methods and call the general class of models “Plug and Play Generative Networks”. PPGNs are composed of (1) a generator network G that is capable of drawing a wide range of image types and (2) a replaceable “condition” network C that tells the generator what to draw. We demonstrate the generation of images conditioned on a class (when C is an ImageNet or MIT Places classification network) and also conditioned on a caption (when C is an image captioning network). Our method also improves the state of the art of Multifaceted Feature Visualization, which generates the set of synthetic inputs that activate a neuron in order to better understand how deep neural networks operate. Finally, we show that our model performs reasonably well at the task of image inpainting. While image models are used in this paper, the approach is modality-agnostic and can be applied to many types of data.
Bio:
Anh Nguyen has completed his Ph.D. study at the University of Wyoming, working with Jeff Clune and Jason Yosinski. His research focus is Deep Learning, specifically understanding and improving deep neural networks. Anh has also worked as a ML research intern at Apple and Uber AI Labs, and a software engineer building front-end user interfaces and visualizations at Bosch and 188bet.com. His research has won Best Paper Awards at CVPR, GECCO, ICML Visualization workshop and Best Research Video Awards at IJCAI and AAAI. His works have also been repeatedly mentioned in MIT Technology Review, Nature, Scientific American and Deep Learning lectures at various institutions.
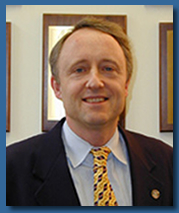
"Imaging the BioMechanical Properties of Tissues"
Abstract:
Significant progress has been made in developing ultrasound and MR techniques that use conventional imaging platforms to sense tissue displacements and calculate the intrinsic biomechanical properties such as the stiffness (Young’s Modulus) and the viscosity. These tissue properties are otherwise hidden yet have high diagnostic value for a wide range of conditions from liver fibrosis to breast cancer. We review the scope of these techniques, including pioneering work done at UR, with an overview of some important clinical applications, and the computational challenges.
Bio:
Kevin J. Parker earned his graduate degrees from MIT and has served at the University of Rochester as Professor, Department Chair, Director of the Rochester Center for Biomedical Ultrasound, and Dean of the School of Engineering and Applied Sciences. His research is in image processing and medical imaging, and he is a fellow of the Institute of Electrical and Electronics Engineers (IEEE), the American Institute of Ultrasound in Medicine (AIUM), the Acoustical Society of America (ASA), and the American Institute for Medical and Biological Engineering (AIMBE). He is an inventor or a founder of a number of enterprises, including the field of elastography and the International Conference series in that area, the Blue Noise Mask, and VirtualScopics, Inc. Professor Parker has over 150 journal publications and dozens of US and international patents..
Tutorials
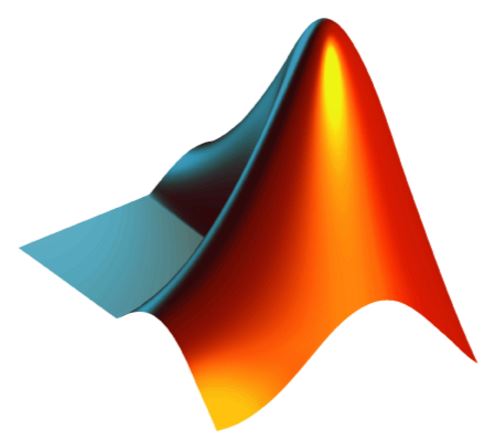
Louvere Walker-Hannon, MathWorks Field Engineer
"Deep Learning for Image Processing & Computer Vision Seminar"
Abstract:
Deep learning techniques have rapidly evolved over the past decade and they are now being used in fields varying from autonomous systems to medical image processing. This session will cover both Machine learning and Deep learning techniques to help solve problems such as object detection, object recognition and classification. This session will cover the following:
- Accessing and managing large data sets
- Machine Learning Techniques for Classification:
We will utilize standard feature extraction methods (SURF, ORB, Bag of Visual Words, etc.) and explore creating a classifier with different techniques (K-NN, SVM, etc.). - Transfer Learning:
An alternative approach to training a model from scratch is using a pre-trained model and re-training to perform a new classification task. We will show how to perform transfer learning in MATLAB. In addition, we will discuss speeding up the training process using GPUs and Parallel Computing Toolbox. - Using a pre-trained CNN as a feature extractor:
We will leverage the use of pre-trained networks for feature extraction. Since the pre-trained network has been trained using many layers and many sample images, the network can be a robust and powerful feature extractor, and we will compare this accuracy to a traditional feature extraction method.
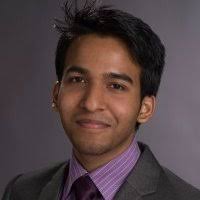
"Tensorflow Tutorial"
Abstract:
TensorFlow is a powerful library for doing large-scale numerical computation. One of the tasks at which it excels is implementing and training deep neural networks. In this session we will learn about:
- TensorFlow's Dataflow Programming Paradigm-
A TensorFlow model creates a directed graph of various computations. In this part we will talk about the pros and cons of this paradigm, which will help you understand the use cases for TensorFlow. - TensorFlow 101-
This section will introduce different APIs available in TensorFlow to implement Convolutional Neural Networks, Sequence to Sequence models and Digital Signal Processing algorithms. - Software Development Workflow-
This part will talk about how to structure a deep learning project and use Docker. - Profiling your code-
In this section you will learn how to profile your code and optimize it to make it run faster. - Multi-GPU execution-
This part will go over distributed training in TensorFlow. - Inference Pipeline-
Lastly, we will show how to deploy your models in mobile devices like Android and the NVIDIA Jetson TX2.
Bio:
Syed Tousif Ahmed is majoring in computer engineering at RIT and works there as a Research Assistant in the National Technical Institute for the Deaf. Syed's interests lie in high performance computing, machine intelligence, digital logic design, and cryptography. Syed worked on several production and startup machine intelligence teams such as NVIDIA, NextDroid LLC, and Ahold USA. He has contributed to several open source frameworks such as TensorFlow, Caffe2 and Torch.
Paper Submission
The Call for Papers can be found here.
Prospective authors are invited to submit a 4-page paper + 5th page of references
here: https://cmt3.research.microsoft.com/WNYISPW2017/Authors should use the same formatting/templates described in the ICIP 2015 Paper Kit.
All accepted papers will be submitted to IEEE Xplore and EI. Past WNYIPW and WNYISPW proceedings can be found here:
Poster Submission
Authors who only want to be considered for a poster presentaiton have the option to submit an abstract in place of a full paper. (Note: Abstract-only submissions will not be searchable on IEEE Xplore)Prospective authors are invited to submit an abstract here: https://cmt3.research.microsoft.com/WNYISPW2017/
Author Attendance
At least one author of each accepted paper or poster must register and attend the workshop to give an oral or poster presentation. Failure to present the paper will result in automatic withdrawal of the paper from being published in the proceedings.Awards
To encourage student participation, a best student paper and best student poster
award will be given. Registration
Registration is available online here. Onsite registration will be also available, with onsite registration fees payable by cash or check. Fees enable attendance to all sessions and include breakfast, lunch, and afternoon snack. Registration fees are:- General Registration: $50 (with online registration by
11/9), $60 (online after 11/9 or onsite)
- Student Registration: $30 (with online registration by 11/9), $40 (online after 11/9 or onsite)
- IEEE or IS&T Membership: $30 (with online registration by 11/9), $40 (online after 11/9 or onsite)
- IEEE or IS&T Student Membership: $20 (with online registration by 11/9), $30 (online after 11/9 or onsite)
Conference at a Glance (Detailed Schedule below)
- 8:30-8:55am, Registration, breakfast
- 8:55-9am, Welcome
- 9-12:30am, Oral presentations
- 9:30am-10:30, Keynote
- 10:30-12:30am, MathWorks deep learning tutorial
- 12:30-2pm, Lunch and posters
- 2-3pm, Keynote
- 3-5pm, Oral presentations
- 3-5pm, Tensorflow tutorial
- 5-5:15pm, Awards
Oral Presentation Instructions
All oral presentations will be 12 minutes long plus 2 minutes for questions. Presentors supply their own laptop with a VGA connector. (Note: there are no HDMI connectors.) Morning/afternoon presentors should test their laptop on the display screen during the 8:15-8:45am or 12:30-1:45pm timeframes respectively. Papers whose first author is a student qualify for best paper award.Poster Presentation Instructions
All printed posters must be no larger than 40" wide x 48" tall. Poster stations will be available for both mounted and unmounted posters. (If you can bring a mounted poster, please do so.) Attachment materials will be provided. All posters must be displayed by 11am and removed by 5:30pm. There are no electrical outlets next to the poster displays. Posters whose first author is a student qualify for best poster award.If your department does not have poster printing capabilities, you can get posters printed at "the Hub Express" in the RIT Student Union, hubxpr@rit.edu, 585-475-3471. Color wide format inkjet is $7/sq.ft. Mounting (up to 30x40) is $4/sq.ft. (Contact the Hub Express if you have larger than 30x40)
Parking Instructions
Any non-RIT attendees are allowed to park in either Lot T or
the Global Village Lot and then walk to Louise Slaughter
Hall (SLA Building). See the
campus map with parking information (you need to print out a parking pass and
place on your windshield). If you forget to print out a permit,
Non-RIT visitors can stop by the RIT Welcome Center (flagpole entrance) on the
day of the Workshop to get a parking pass.Tim Mathieu, MathWorks Field Engineer
Louvere Walker-Hannon, MathWorks Field Engineer
"Deep Learning for Image Processing & Computer Vision Seminar"
Detailed Schedule
- 8:30-8:55am, Registration, breakfast (Rooms 2210-2240)
- 8:55-9am, Welcome by Conference Chair (Rooms 2210-2240)
- 9am-12:30pm, Oral presentations (Rooms 2210-2240)
- 9am: Invited talk: "On-Device Intelligence : Are we there yet? ", Dhireesha Kudithipudi, Professor, Rochester Institute of Technology
- 9:30am: Keynote talk: "Egocentric Computer Vision, for Fun and for Science", David J. Crandall, Associate Professor, Indiana University
- 10:20am: AM break
- 10:30am-11:30am: Oral papers
- “Using Road Markers as Fiducials for Automatic Speed Estimation in Road Videos”, D. Kamat, T. Kinsman.
- “Deep Learning for Philately Understanding”, R. Dhamdhere, T. Nguyen, L. Rausch, R. Ptucha.
- “Self Interference Cancellation for Bandwidth Optimization on Satellite Communications”, A. Marseet, F. Sahin.
* “Multistream Hierarchical Boundary Network for video captioning”, T. Nguyen, S. Sah, R. Ptucha. - 11:45am: Invited talk: “Plug & Play Generative Networks: Conditional Iterative Generation of Images in Latent Space”, Anh Nguyen, Assistant Professor, University of Auburn
- 10:30am-12:30pm, MathWorks Tutorial (Room 2120): “Deep Learning for Image Processing & Computer Vision”
- Louvere Walker-Hannon, MathWorks Field Engineer
- Tim Mathieu, MathWorks Field Engineer
- 12:30-2pm, Lunch and poster displays (Rooms 2210-2240, overflow 2140)
- “Systolic Architectures for Recurrent Neural Networks”, S. Ahmed.
- “Colour Contrast Detection in Chromaticity Diagram: A new Computerized Colour Vision Test”, I. Benkhaled, I. Marc, D. Lafon.
- “Quantitative Approach to Assessing Stress in Autistic Individuals”, S. Ahmed, E. Coppola, M. Nixt, J. Strinka, E. Gioe, J. Amerault.
- “Spectrogram Video Segmentation for Multi Instrument Detection from a Single Source”, D. Luong.
- “A New Approach for Detecting Copy-Move Forgery in Digital Images”, H. Shabanian, F. Mashhadi.
** “Measuring Catastrophic Forgetting in Neural Networks”, R. Kemker, M. McClure, A. Abitino, T. Hayes, C. Kanan. - “An analysis of visual question answering algorithms”, K Kafle, C. Kanan.
- “Image Processing used for the Recognition and Classification of Coin-Type Ancient Artifacts”, V. Tompa, M. Dragomir, D. Hurgoiu, C. Neamtu.
- “Application of Complex-Valued Convolutional Neural Network for Next Generation Wireless Networks”, A. Marseet, F. Sahin.
- “Collaborative Filtering Based Recommender System for Commercial Optimization”, S. Gopalakrishnan.
- “Iris Recognition using Low Fidelity Images”, K. Prasanna Simha, A. Bhat, S. Jain.
- “Automatic Trellis Generation for Demodulation of Faster Than Nyquist Signals”, D. Govindaraj, M. Bazdresch.
- 2-5pm, Oral presentations (Rooms 2210-2240)
- 2pm, Keynote: “Efficient Training and Inference of Very Deep Convolutional Networks”, Gao Huang, Postdoctoral Fellow in the Department of Computer Science at Cornell University
- 2:50pm: PM break
- 3pm: Invited talk: "Imaging the BioMechanical Properties of Tissues", Kevin Parker, May Professor of ECE, Dean Emeritus, University of Rochester
- 3:30pm: Invited talk: “Deep Discriminative and Generative Models for Temporal Multimodal Data Fusion”, Dr. Edgar A. Bernal, United Technologies Research Center
- 4pm-4:50pm: Oral papers
- “Source-Separated Audio Input for Accelerating Convolutional Neural Networks”, M. Dominguez, M. Daigneau, R. Ptucha.
- “Analysis of Noisy 2D Angiographic Images for Improved Blood Flow Rate Quantification in Dialysis Access”, N. Koirala, G. McLennan.
- “Multi-Scale Morphological Analysis for Retinal Vessel Detection In Wide-Field Fluorescein Angiography”, L. Ding, A. Kuriyan, R. Ramchandran, G. Sharma.
- “Non-Native Phragmites Colony Detection System”, D. Sharma.
- 4:40-5pm: PM break (Committee meeting to select best paper and best poster)
- 3-5pm, Tensorflow Tutorial (Room 2140):
- Syed Ahmed, Student and Researcher, Rochester Institute of Technology
- 5-5:15pm, Closing and Awards (Rooms 2210-2240)
* MathWorks Best Student Paper Award.
** NVIDIA Best Student Poster Award.
Organizing Committee
- Ziya Arnavut, SUNY Fredonia
- Nathan Cahill, Rochester Institute of Technology
- Zhiyao Duan, University of Rochester
- Christopher Kanan, Rochester Institute of Technology
- Paul Lee, University of Rochester
- Cristian Linte, Rochester Institute of Technology
- David Odgers, Odgers Imaging
- Raymond Ptucha, Rochester Institute of Technology
- Richard Zanibbi, Rochester Institute of Technology