ICCRE 2020 Plenary Speakers
Prof. Juntao Fei (费俊涛教授)
Hohai University, China (中国河海大学)
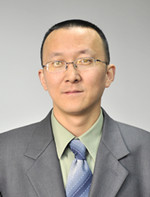
Biography: Juntao Fei is now a professor at the College of IoT Engineering, Hohai University , Director of Institute of Electrical and Control Engineering He received his B.S. degree from the Hefei University of Technology, M.S. degree from University of Science and Technology of China, M.S and Ph.D. degree from the University of Akron, USA. He was a visiting scholar at University of Virginia, USA, North Carolina State University, USA respectively. He served as an assistant professor at the University of Louisiana, USA from 2007 to 2009.
He is a Senior Member of IEEE. He has actively served in the editorial board of a number of international journals as an associate editor, including Transactions of the Institute of Measurement and Control, IEEE Access, etc. He has served as chairs and program committee members for numerous international conferences. He was a PI of 30 projects in the last ten years, including the National Natural Science Foundation of China, Jiangsu Natural Science Foundation, etc. He has published more than 300 journal and conference papers and five books , more than 130 papers were published by international journals such as IEEE Trans. on Cybernetics, IEEE Trans. on Neural Network and Learning System , IEEE Trans. on Power Electronics, IEEE Transactions on Control Systems Technology, IEEE Transactions on Systems, Man, and Cybernetics: Systems, etc. He authorized 92 invention patents. He is an awardee of the Recruitment Program of Global Experts (China). His biography has been included in Who’s Who in the World, Who’s Who in Science and Engineering, Who’s Who in America.
His current research interests are adaptive control, intelligent control, mechatronics and robotics, power electronics and control, smart materials and structure.
Title of Speech: Novel Adaptive Neural Network Sliding Mode Controller of Active Power Filter
Abstract: This speech presents an adaptive sliding mode controller based on a novel double hidden layer recurrent neural network algorithm to improve the performance of harmonic current compensation and robustness of an active power filter. The double hidden layer neural network can improve the accuracy and generalization ability of the neural network, reduce the number of network weights and accelerate the network training speed owing to the strong fitting and presentation ability of two-layer activation functions. The feedback neural network plays the significant role in possessing associative memory and rapid system convergence. Simulation and experimental results demonstrated that the satisfactory performance of proposed controller under both dynamic and steady state operations, including robustness, fast response, and small overshoot for harmonic suppression.
Prof. Ben Abdallah Abderazek
University of Aizu, Japan
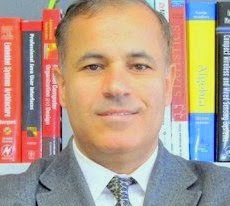
Biography: Abderazek Ben Abdallah is a full Professor of computer science and engineering and the Head of the Division of Computer Engineering, The University of Aizu, Aizu-Wakamatsu, Japan, since April 2014. He has been a faculty member at the University of Aizu since 2007. Before joining the University of Aizu, he was a Research Associate with the Graduate School of Information Systems (now Graduate School of Informatics and Engineering), The University of Electro-Communications, Tokyo, from 2002 to 2007. He held several visiting professorships at several universities, including The Hong Kong University of Science and Technology, Hong Kong (2010-2013), and Huazhong University of Science and Technology, Wuhan (2010-2016). He received his Ph.D. degree in computer engineering from the University of Electro-Communications, Tokyo, in 2002, his M.S degree in computer engineering, and his B.S. degree in electrical engineering from Hauzhong University of Science and Technology, in 1997 and 1994, respectively. Prof. Ben Abdallah’s primary research interests include computer architecture, adaptive/self-organizing systems, power and reliability-aware architectures, network on chips (photonic, hybrid), fault-tolerance, and neuro-inspired/neuromorphic computing. Most recently, his research group has been building scalable, large-scale, and energy-efficient neuromorphic processing systems using application-specific hardware and embedded reconfigurable systems. He has authored four books, published more than 150 journal articles and conference papers, and holds several patents in these areas. He is the recipient of the President’s prize for scientific research and technology in 2010 and five best paper awards. He is a Senior Member of IEEE and ACM. He served on the chair, editorial, and review boards of several journals and conferences including, founder and steering chair of the IEEE MCSoC symposium series. He has also been involved in co-organizing many symposia, and conferences as well as guest editor of special issues in journals, such as IEEE Transactions on Emerging Topics in Computing.
Title of Speech: Neuromorphic Computing: Beyond-CMOS Approach to Future Computing
Abstract: Recently, neuroscience research has revealed a great deal about the structure and operation of individual neurons, and therapeutic tools have also shown a great deal about how neural activity in the different regions of the brain follows a sensory stimulus. Moreover, the advances of software-based AI have brought us to the edge of building low-power neuromorphic processors with cognitive behavior. Hardware implementations of neuromorphic chips have the advantage of computational speedup of machine-learning applications over software implementation and can take full advantage of their inherent parallelism. The need for no latency, higher security, faster computing, and less dependence on connectivity will drive the adoption of devices that can offer AI at the edge to run machine learning algorithms more efficiently. However, edge IoT and consumer endpoint devices need high-performance inference processing at low cost in power, price, and die size. The first part of this talk presents current fundamental trends, AI-chips design principles, and their potential applications. The second part discusses several case studies of neuromorphic chips design developed by the speaker’s research group. The last section of the talk describes prospects on AI-chips and their impacts on future computing. I will emphasize on how neuromorphic applications can provide solutions to the challenges of machine learning, including latency, power consumption, and security.