2017 IEEE Symposium on Adaptive Dynamic Programming and Reinforcement Learning (IEEE ADPRL'17)
Adaptive dynamic programming (ADP) and reinforcement learning (RL) are two related paradigms for solving decision making problems where a performance index must be optimized over time. ADP and RL methods are enjoying a growing popularity and success in applications, fueled by their ability to deal with general and complex problems, including features such as uncertainty, stochastic effects, and nonlinearity.
ADP tackles these challenges by developing optimal control methods that adapt to uncertain systems over time. A user-defined cost function is optimized with respect to an adaptive control law, conditioned on prior knowledge of the system and its state, in the presence of uncertainties. A numerical search over the present value of the control minimizes a nonlinear cost function forward-in-time providing a basis for real-time, approximate optimal control. The ability to improve performance over time subject to new or unexplored objectives or dynamics has made ADP successful in applications from optimal control and estimation, operation research, and computational intelligence.
RL takes the perspective of an agent that optimizes its behavior by interacting with its environment and learning from the feedback received. The long-term performance is optimized by learning a value function that predicts the future intake of rewards over time. A core feature of RL is that it does not require any a priori knowledge about the environment. Therefore, the agent must explore parts of the environment it does not know well, while at the same time exploiting its knowledge to maximize performance. RL thus provides a framework for learning to behave optimally in unknown environments, which has already been applied to robotics, game playing, network management and traffic control.
The goal of the IEEE Symposium on ADPRL is to provide an outlet and a forum for interaction between researchers and practitioners in ADP and RL, in which the clear parallels between the two fields are brought together and exploited. We equally welcome contributions from control theory, computer science, operations research, computational intelligence, neuroscience, as well as other novel perspectives on ADPRL. We host original papers on methods, analysis, applications, and overviews of ADPRL. We are interested in applications from engineering, artificial intelligence, economics, medicine, and other relevant fields.
Topics
Specific topics of interest include, but
are not limited to:
- Convergence and performance analysis
- RL and ADP-based control
- Function approximation and value function representation
- Complexity issues in RL and ADP
- Policy gradient and actor-critic methods
- Direct policy search
- Planning and receding-horizon methods
- Monte-Carlo tree search and other Monte-Carlo methods
- Adaptive feature discovery
- Parsimoneous function representation
- Statistical learning and PAC bounds for RL
- Learning rules and architectures
- Bandit techniques for exploration
- Bayesian RL and exploration
- Finite-sample analysis
- Partially observable Markov decision processes
- Neuroscience and biologically inspired control
- ADP and RL for multiplayer games and multiagent systems
- Distributed intelligent systems
- Multi-objective optimization for ADPRL
- Transfer learning
- Applications of ADP and RL
- Deep learning combined with ADPRL
Accepted Special Sessions
- Novel Distributed Adaptive Dynamic Programming and Reinforcement Learning Designs for Networked Multi-Agent Systems
- Organizers:
Hao Xu, University of Nevada, NV, USA;
Zhen Ni, South Dakota State University, SD, USA;
Avimanyu Sahoo, Oklahoma State University, OK, USA - More Information
- Adaptive Dynamic Programming and Reinforcement Learning for Smart Power Grid and Sustainable Energy Systems
- Organizers:
Zhen Ni, South Dakota State University, SD, USA
Xiangjun Li, China Electric Power Research Institute, Beijing, China
Qinglai Wei, Chinese Academy of Sciences, Beijing, China
Hao Xu, University of Nevada, Reno, NV, USA - More Information
- Reinforcement learning and inverse reinforcement learning for average reward case
- Organizers:
Xuesong Wang, China University of Mining and Technology, China
Yang Gao, Nanjing University, China
Yanjie Li, Harbin Institute of Technology, Shenzhen, China
Chunlin Chen, Nanjing University, China
Yuhu Cheng, China University of Mining and Technology, China - More Information
- Data-driven/Data-based Adaptive Dynamic Programming for Uncertain Nonlinear Systems
- Organizers:
Qichao Zhang, Chinese Academy of Sciences, Beijing, China
Ding Wang, Chinese Academy of Sciences, Beijing, China
Chaoxu Mu, Tianjin University, Tianjin, China - More Information
- Learning and Adaptation in Cyber-Physical Systems
- Organizers:
K. G. Vamvoudakis, Kevin T. Crofton Department of Aerospace and Ocean Engineering, Virginia, USA
F. L. Lewis, University of Texas at Arlington, USA - More Information
Symposium Co-Chairs
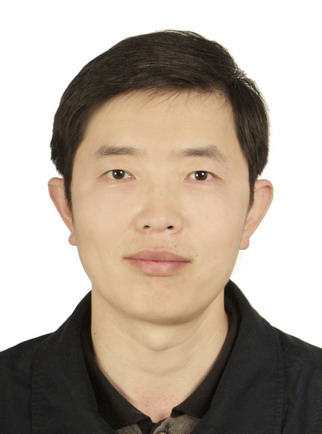
Dongbin Zhao
Chinese Academy of Sciences, China.
Email: dongbin.zhao@ia.ac.cn
Jagannathan Sarangapani
Missouri University of Science and Technology, USA
Email: sarangap@mst.edu