2017 IEEE Symposium
on Deep Learning (IEEE DL'17)
Deep Learning (DL)
is growing in popularity because it exploits rather well the
unreasonable effectiveness of data to solve complex problems in machine
learning. In fact, multi scale machine perception tasks such as object
and speech recognitions using DL have recently outperformed systems
that have been under development for many years. The principles of DL,
and its ability to capture multi scale representations, are very
general and the technology can be applied to many other problem
domains, which makes it quite attractive. Sponsored by the IEEE
Computational Intelligence Society, this event will attract top
scientists, researchers, professionals, practitioners and students from
around the world.
Topics
The
goal of the IEEE Symposium on DL is to provide a forum for interactions
between researchers and practitioners in DL as well as in Artificial
Neural Networks, Bayesian Learning, Generative and Predictive Modeling,
Optimization, Cognitive Architectures and Machine Learning with an
interest in DL. We are interested in discussing the new DL advances,
the challenges ahead, and to brainstorm about new solutions and
directions. We also seek applications from large engineering firms
dedicated to construction and services in energy, autonomous
transportation, communications industries, web, marketing, medical and
financial services, and scientific fields that require big data
analytics.
Topics of IEEE DL’17 include but are not limited to:
Topics of IEEE DL’17 include but are not limited to:
- Unsupervised, semi-supervised, and supervised learning
- Deep reinforcement learning (deep value function estimation, policy learning and stochastic control)
- Memory Networks and differentiable programming
- Implementation issues, both software and hardware platforms
- Applications in vision, audio, speech, natural language processing, robotics, navigation, control, games AI, cognitive architectures, etc.
- Dimensionality expansion and sparse modeling
- Learning representations from large-scale data
- Multi-task learning
- Learning from multiple modalities
- Weakly supervised learning
- Metric learning and kernel learning
- Hierarchical models
- Paralleliisation in DL
- Non-Iterative DL
- Recursive DL
- Incremental DL
- Evolving DL
- Fast DL
Accepted
Special Sessions
Symposium Co-Chairs
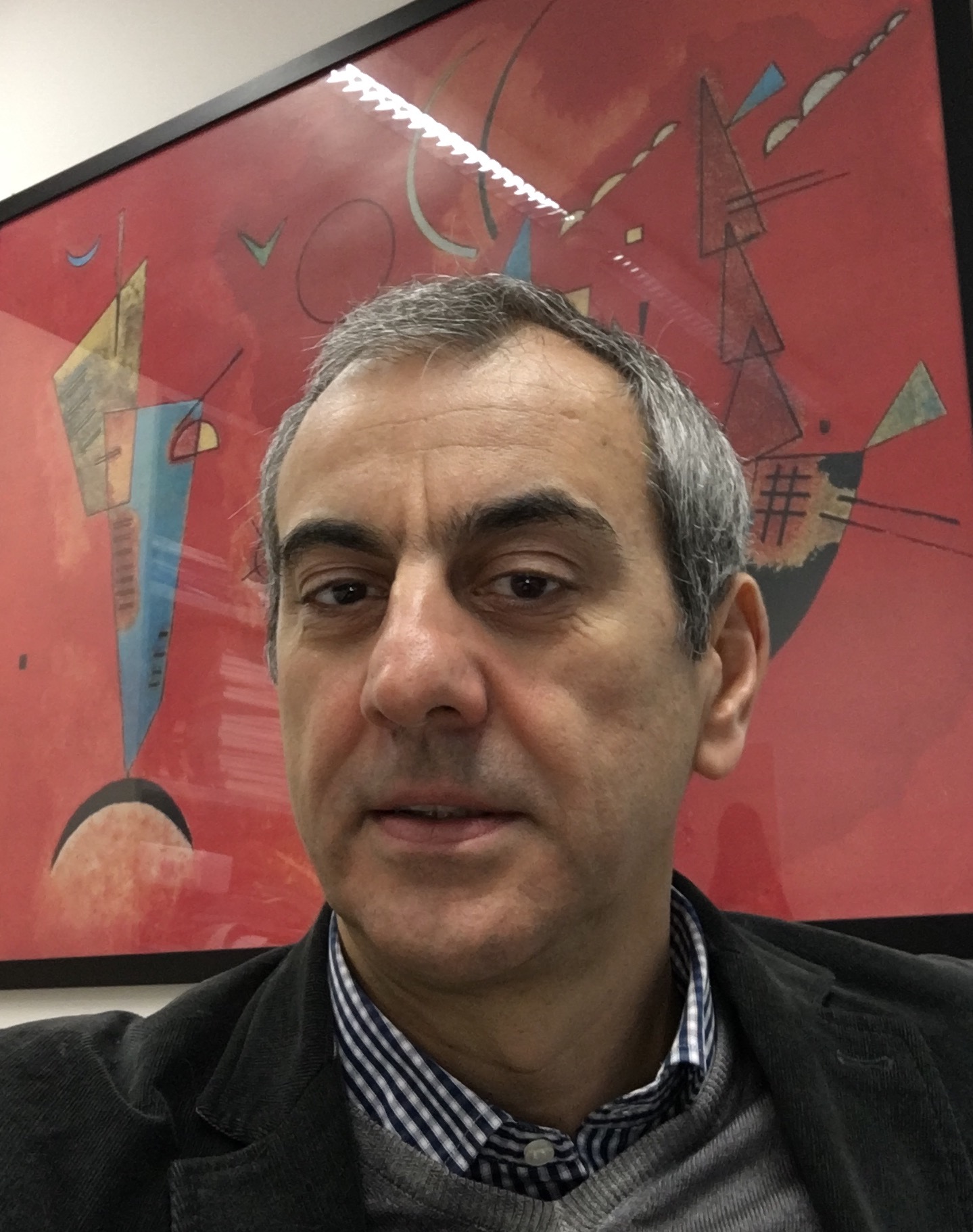
Alessandro Sperdutii
Università di Padova, Italy
Email: sperduti@math.unipd.it
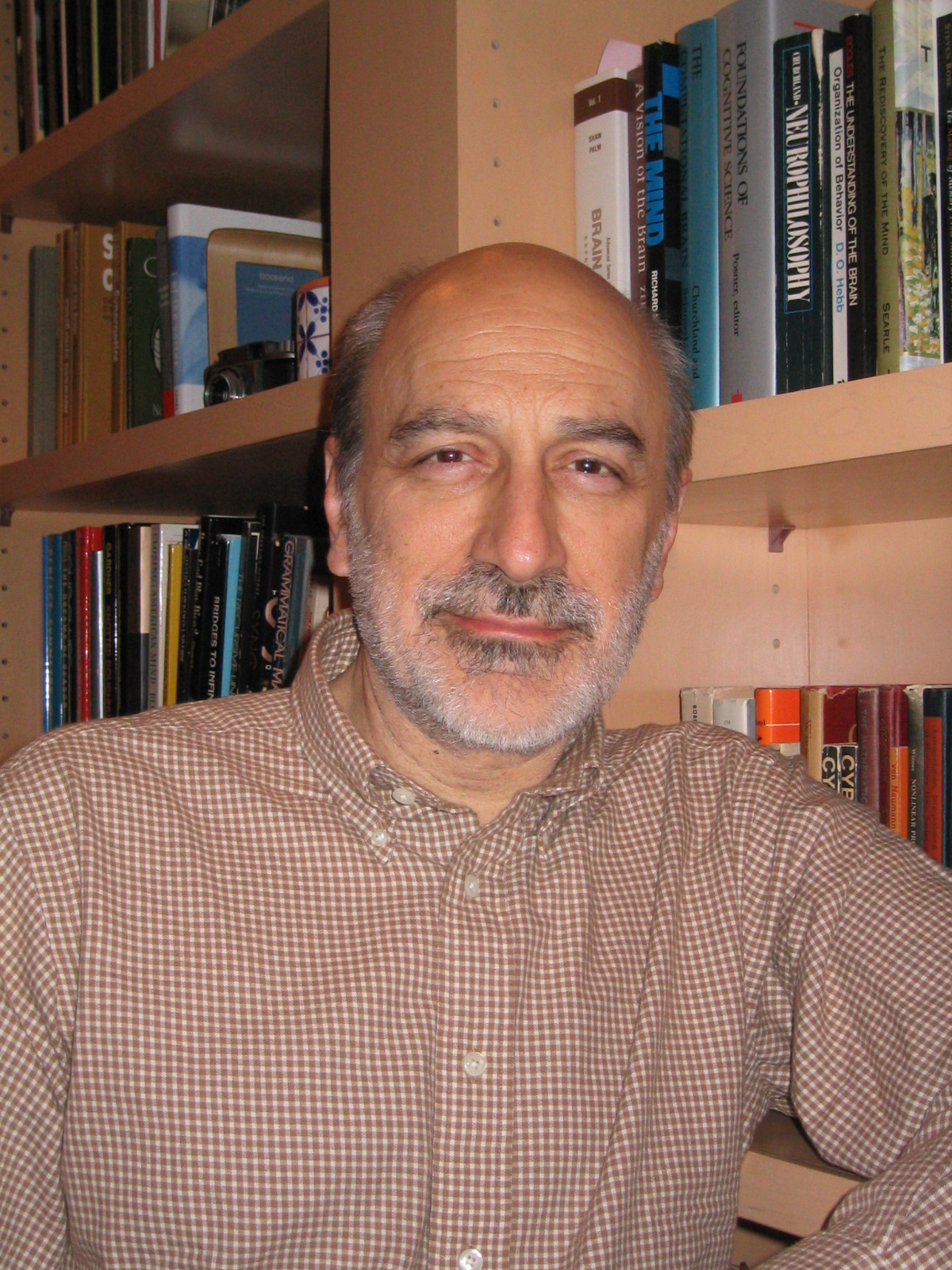
Jose Principe
University of Florida, USA
Email: principe@cnel.ufl.edu

Plamen Angelov
University of Lancaster, UK
Email: p.angelov@lancaster.ac.uk
Program
Committee
- Akihito Sudo, Tokyo University, Japan
- Aldy Gunawan, Singapore Management Univ, Singapore
- Alessandro Sperduti, University of Padova, Italy
- Amit Sethi, Indian Inst.of Technology Guwahati, India
- Arijit Sur, Indian Inst.of Technology Guwahati, India
- Ashish Anand, Indian Inst. Technology Guwahati, India
- Chrisina Jayne, Robert Gordon University, UK
- Dmitry Kangin, Exeter University, UK
- Erdal Kayacan, Nanyang Technological Univ., Singapore
- Feng Yuhong, Shenzhen University, China
- Huang Guang Bin, Nanyang Technological Univ, Singapore
- Jose C. Principe, University of Florida, US
- Justin Dauwels, Nanyang Technological Univ., Singapore
- Olga Senyukova, Lomonosov Moscow State Univ., Russia
- Teck-Hou Teng, Singapore Management Univ., Singapore
- Wang Lipo, Nanyang Technological Univ., Singapore
- Wang Di, Nanyang Technological Univ., Singapore
- William Howell, Natural Resources Canada